Goods source optimal allocation model construction method, optimal allocation model and optimal allocation method
A technology for building methods and models, applied in the field of deep learning, to achieve the effect of assisting decision-making, reducing human operation errors, and accurate supply of goods
- Summary
- Abstract
- Description
- Claims
- Application Information
AI Technical Summary
Problems solved by technology
Method used
Image
Examples
Embodiment 1
[0049] A method for constructing a supply optimization model of the present invention includes the following steps:
[0050] S100, constructing a BP neural network model and an LSTM cyclic neural network model, the BP neural network model is used for data feature learning on retailer basic information data, and the LSTM cyclic neural network model is used for circulating retailer historical order data long-term memory learning;
[0051] Collect basic retailer information data and retailer historical order data, and divide retailer basic information data and retailer historical order data into test samples and training samples;
[0052]S200. Taking the retailer's basic information data in the training sample as the first-level input parameter, training the BP neural network model model through the first-level input parameter, outputting the retailer's supply history distribution data, and obtaining the trained BP neural network model;
[0053] Taking the output historical dist...
Embodiment 2
[0069] An optimal matching model of goods sources according to the present invention, which is a model constructed by a method for constructing an optimal matching model of goods sources disclosed in Embodiment 1, including a trained BP neural network model and a trained LSTM cyclic neural network model.
[0070] After training, the BP neural network model is used to learn the data characteristics of the retailer's basic information data. After the training, the LSTM cycle neural network model is used to perform cyclic long-term memory learning on the retailer's historical order data. After the above training, the BP neural network model and training The post-LSTM cycle neural network model obtains the historical distribution data of retailer's supply.
[0071] In this embodiment, both the BP neural network model and the LSTM cyclic neural network model include an input layer, a hidden layer and an output layer. There are five hidden layers in the BP neural network model, and ...
Embodiment 3
[0073] A method for optimal matching of goods sources based on deep learning of the present invention comprises the following steps:
[0074] S100. Using the method for constructing an optimized supply matching model disclosed in Embodiment 1, construct an optimal matching model for supply sources, the model including a trained BP neural network model and a trained LSTM cyclic neural network model;
[0075] S200. Collect retailer basic information data and retailer historical order data;
[0076] S300, using the retailer's basic information data as a first-level input parameter, and inputting the first-level input parameter into the trained BP neural network model to obtain the retailer's supply history distribution data;
[0077] The historical distribution data of the retailer's supply of goods and the historical ordering data of the retailer are used as the secondary input parameters, and the secondary input parameters are input into the trained LSTM cyclic neural network m...
PUM
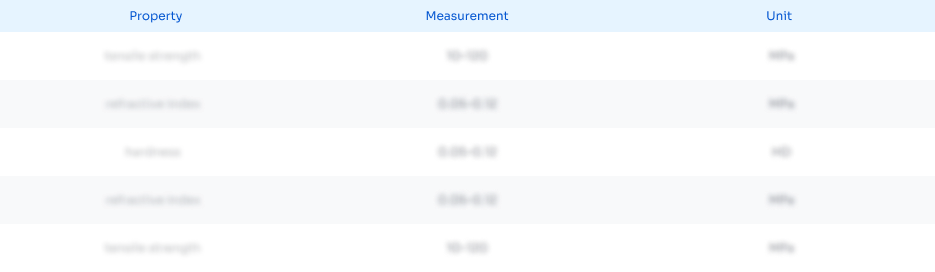
Abstract
Description
Claims
Application Information
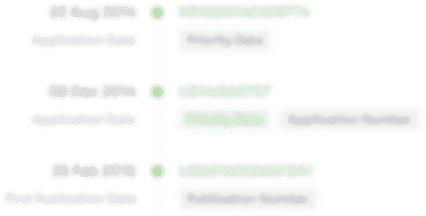
- R&D Engineer
- R&D Manager
- IP Professional
- Industry Leading Data Capabilities
- Powerful AI technology
- Patent DNA Extraction
Browse by: Latest US Patents, China's latest patents, Technical Efficacy Thesaurus, Application Domain, Technology Topic, Popular Technical Reports.
© 2024 PatSnap. All rights reserved.Legal|Privacy policy|Modern Slavery Act Transparency Statement|Sitemap|About US| Contact US: help@patsnap.com