Semantic segmentation method based on multi-scale deformable convolution
A semantic segmentation, multi-scale technology, applied in instruments, biological neural network models, character and pattern recognition, etc., can solve the problem of not being able to extract spatial information well
- Summary
- Abstract
- Description
- Claims
- Application Information
AI Technical Summary
Problems solved by technology
Method used
Image
Examples
Embodiment Construction
[0022] In order to make the technical solution of the present invention clearer, the present invention will be further elaborated below in conjunction with the accompanying drawings. The present invention is concretely realized according to the following steps:
[0023] The first step is to prepare the dataset.
[0024] Collect images of different categories, and after unifying the categories, generate image label information for the selected category. Each label image is single-channel, and the value of each pixel corresponds to the selected category. The collected images are divided into training set, verification set and test set. The training set is used to train the convolutional neural network, the verification set is used to select the best training model, and the test set is used for subsequent testing of the model effect or practical application. For ease of use, this patent uses the public PASCALVOC2012 data set for related experiments.
[0025] The second step is ...
PUM
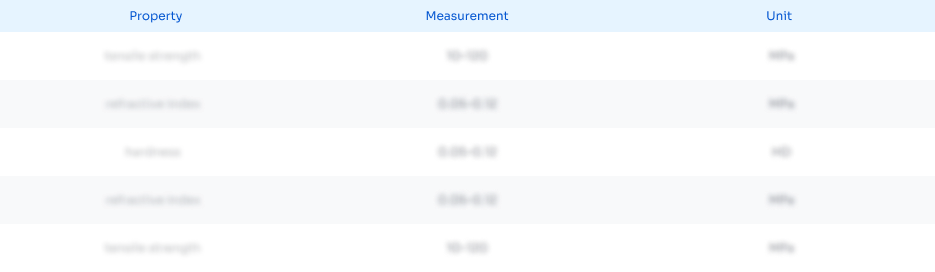
Abstract
Description
Claims
Application Information
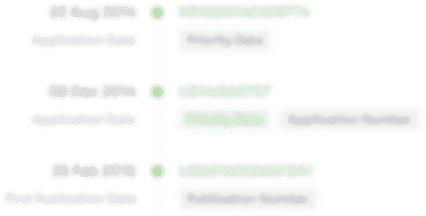
- R&D
- Intellectual Property
- Life Sciences
- Materials
- Tech Scout
- Unparalleled Data Quality
- Higher Quality Content
- 60% Fewer Hallucinations
Browse by: Latest US Patents, China's latest patents, Technical Efficacy Thesaurus, Application Domain, Technology Topic, Popular Technical Reports.
© 2025 PatSnap. All rights reserved.Legal|Privacy policy|Modern Slavery Act Transparency Statement|Sitemap|About US| Contact US: help@patsnap.com