Radar interference signal feature-level fusion identification method based on deep convolutional neural network
A convolutional neural network and radar jamming technology, which is applied in the field of radar jamming signal feature-level fusion recognition, can solve problems such as features that are easily affected by noise and redundancy, and achieve the effect of reducing impact and facilitating design.
- Summary
- Abstract
- Description
- Claims
- Application Information
AI Technical Summary
Problems solved by technology
Method used
Image
Examples
specific Embodiment approach 1
[0042] Specific implementation mode 1. Combination figure 1 and figure 2 As shown, the present invention provides a radar jamming signal feature level fusion recognition method based on a deep convolutional neural network, including establishing a radar jamming time domain data set, and using two types of radar jamming time domain data in the radar jamming time domain data set The feature vectors are extracted in different forms, and then the two extracted feature vectors are fused in series; the fused feature vectors are used to train the support vector machine, and the trained radar jamming signal feature-level fusion recognition model is obtained;
[0043] The two kinds of feature vectors extracted include: feature vectors extracted by using a one-dimensional convolutional neural network and expert feature vectors extracted manually;
[0044] Or use the feature vector extracted by a one-dimensional convolutional neural network and the time-frequency domain feature vector ...
specific Embodiment 1
[0050] to combine figure 1 As shown, for the eigenvector extracted by one-dimensional convolutional neural network and the expert eigenvector extracted manually, the process of obtaining the feature-level fusion recognition model of radar jamming signal includes:
[0051] Artificial feature parameter extraction is performed on the radar interference time domain data, and the obtained expert feature vector includes time domain moment skewness, time domain moment kurtosis, time domain signal envelope fluctuation, time domain interference signal mean and time domain interference signal variance ;
[0052] At the same time, the radar interference time domain data is divided into training set, verification set and test set;
[0053] The data in the training set is extracted using a one-dimensional convolutional neural network to extract the training sample feature vector, and the training sample feature vector is subjected to PCA processing, and then the PCA-processed feature vect...
specific Embodiment 2
[0072] combine figure 2 As shown, for the feature vectors extracted by using one-dimensional convolutional neural network and the time-frequency domain feature vectors extracted by using deep convolutional neural network, the process of obtaining the feature-level fusion recognition model of radar jamming signals includes:
[0073] Divide radar jamming time domain data into time domain training set, time domain validation set and time domain test set;
[0074] Use one-dimensional convolutional neural network to extract time-domain training sample feature vectors for the data in the time-domain training set;
[0075] At the same time, time-frequency transformation is performed on the radar interference time-domain data to obtain radar interference time-frequency domain data, and the radar interference time-frequency domain data is divided into time-frequency domain training set, time-frequency domain verification set and time-frequency domain test set;
[0076] The time-frequ...
PUM
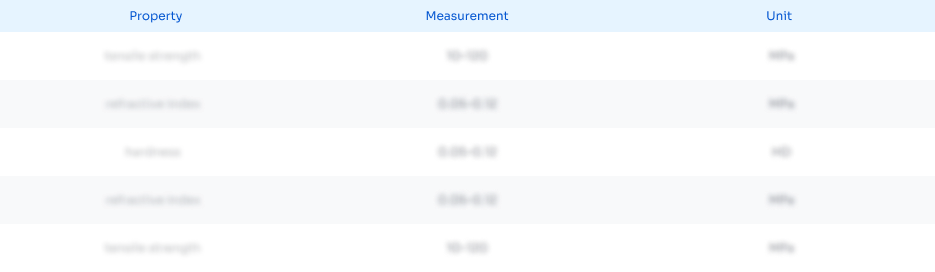
Abstract
Description
Claims
Application Information
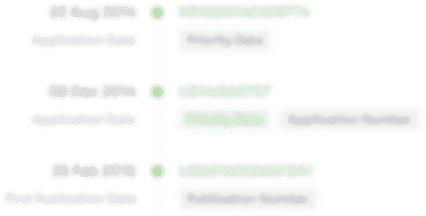
- R&D
- Intellectual Property
- Life Sciences
- Materials
- Tech Scout
- Unparalleled Data Quality
- Higher Quality Content
- 60% Fewer Hallucinations
Browse by: Latest US Patents, China's latest patents, Technical Efficacy Thesaurus, Application Domain, Technology Topic, Popular Technical Reports.
© 2025 PatSnap. All rights reserved.Legal|Privacy policy|Modern Slavery Act Transparency Statement|Sitemap|About US| Contact US: help@patsnap.com