Piloted driving vehicle training method based on virtual environment and depth double-Q network
A virtual environment and automatic driving technology, applied in the direction of probability network, neural learning method, based on specific mathematical models, etc., can solve the problems of poor robustness, labor-intensive data collection, and variation, etc., to achieve strong robustness, The training process is fast and stable, avoiding a large amount of workload and the effect of high requirements for human manipulation
- Summary
- Abstract
- Description
- Claims
- Application Information
AI Technical Summary
Problems solved by technology
Method used
Image
Examples
Embodiment Construction
[0039] The specific implementation manner of the present invention will be described below with the algorithm steps in conjunction with the accompanying drawings, so that those skilled in the art can better understand the present invention.
[0040] The present invention proposes a self-driving car training method based on a virtual environment and a deep double-Q network, such as figure 1 shown in the following steps:
[0041] Step 1: Refer to the real track, preset the environmental parameters, and build a virtual environment for the car track suitable for reinforcement learning training based on Unity;
[0042] In this embodiment, run under the Linux system, download and configure Unity and OpenAI gym. Using the game engine sandbox in Unity, according to the size of the physical car, set the road property to a two-way street with a width of 60cm. The size of the car in the virtual environment is set to a ratio of 1:16. The frame skipping parameter in the Unity environment ...
PUM
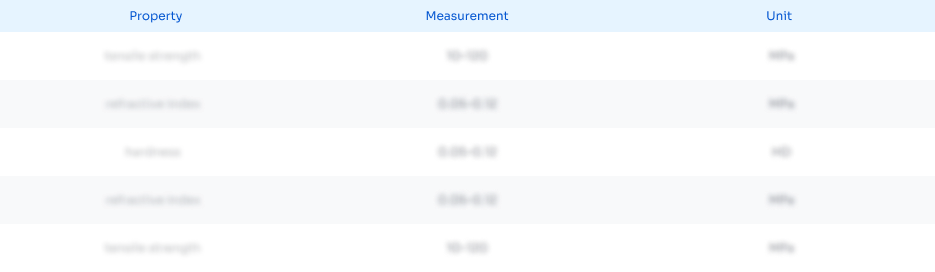
Abstract
Description
Claims
Application Information
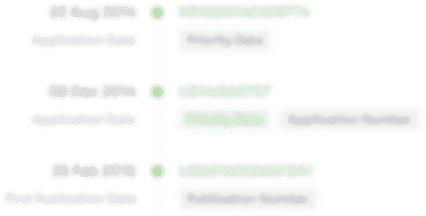
- R&D Engineer
- R&D Manager
- IP Professional
- Industry Leading Data Capabilities
- Powerful AI technology
- Patent DNA Extraction
Browse by: Latest US Patents, China's latest patents, Technical Efficacy Thesaurus, Application Domain, Technology Topic, Popular Technical Reports.
© 2024 PatSnap. All rights reserved.Legal|Privacy policy|Modern Slavery Act Transparency Statement|Sitemap|About US| Contact US: help@patsnap.com