Cooperative agent learning method based on multi-agent reinforcement learning
A multi-agent, reinforcement learning technology, applied in the field of machine learning, can solve problems such as poor collaboration and low efficiency, and achieve the effects of improving efficiency, stable model training, and simplifying model complexity
- Summary
- Abstract
- Description
- Claims
- Application Information
AI Technical Summary
Problems solved by technology
Method used
Image
Examples
Embodiment
[0039] Such as Figure 1-3 Shown is a kind of learning method of cooperative agent based on multi-agent reinforcement learning, comprising the following steps:
[0040] Step 1: Reset multiple target environments, which meet the characteristics of multi-agents in a cooperative relationship sharing information and acting together;
[0041] Step 2: Initialize the policy network π θ The model parameter θ of π and global information prediction network f θ The model parameter θ of f ;
[0042] Step 3: Parallel sampling of multi-agents in multiple environments with the current strategy π in the environment with a fixed number of steps; in each step, the environment e i Multiple agents in share the same state S i,t , to extract the global feature s for this state i,t,global , and for each agent pair state s i,t Extract local features, and combine them to obtain agent features s i,t,comb Finally, it is used as the input data of the policy network model;
[0043] The agents in...
PUM
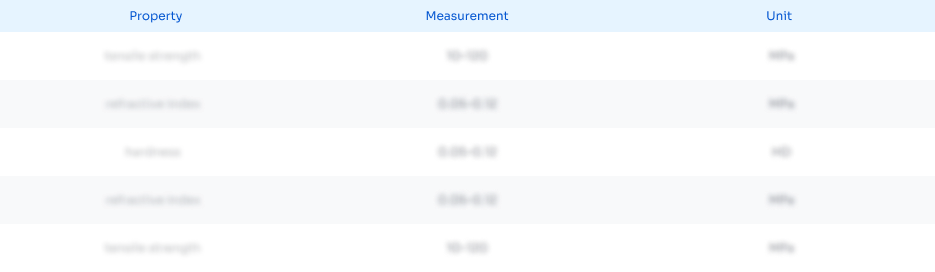
Abstract
Description
Claims
Application Information
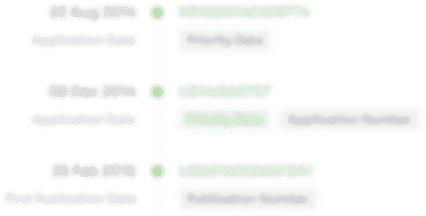
- Generate Ideas
- Intellectual Property
- Life Sciences
- Materials
- Tech Scout
- Unparalleled Data Quality
- Higher Quality Content
- 60% Fewer Hallucinations
Browse by: Latest US Patents, China's latest patents, Technical Efficacy Thesaurus, Application Domain, Technology Topic, Popular Technical Reports.
© 2025 PatSnap. All rights reserved.Legal|Privacy policy|Modern Slavery Act Transparency Statement|Sitemap|About US| Contact US: help@patsnap.com