Knowledge representation method for cross-media knowledge reasoning task
A technology of knowledge reasoning and knowledge representation, which is applied in the field of knowledge representation for cross-media knowledge reasoning tasks, can solve the problem of low reasoning accuracy, and achieve the effect of improving discrimination ability and accuracy
- Summary
- Abstract
- Description
- Claims
- Application Information
AI Technical Summary
Problems solved by technology
Method used
Image
Examples
Embodiment
[0046] As mentioned above, a knowledge representation method for cross-media knowledge reasoning tasks includes the following steps:
[0047] 1) Triple extraction of cross-media knowledge graph: extract the data in the existing cross-media knowledge graph and convert it into RDF triple form;
[0048] Specifically, due to the different carriers of knowledge storage in cross-media knowledge graphs, the mainstream is to use RDF triples as the carrier of knowledge storage, and some use relational databases as the carrier of knowledge storage. The knowledge stored in relational databases can be Use D2R tools to convert to RDF triples;
[0049] 2) Data preprocessing: Extract the head entity, relationship and tail entity information of RDF triples from the statistical cross-media knowledge map, save the entity information and relationship information respectively, remove duplicate entity and relationship data, and additionally filter unnecessary Entities and relationships that confo...
PUM
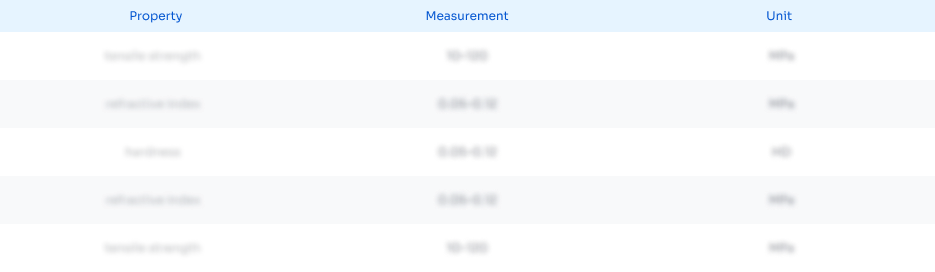
Abstract
Description
Claims
Application Information
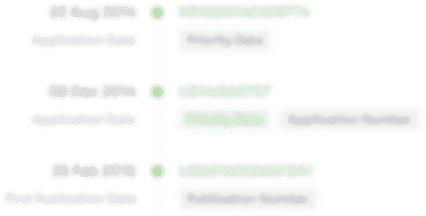
- R&D Engineer
- R&D Manager
- IP Professional
- Industry Leading Data Capabilities
- Powerful AI technology
- Patent DNA Extraction
Browse by: Latest US Patents, China's latest patents, Technical Efficacy Thesaurus, Application Domain, Technology Topic.
© 2024 PatSnap. All rights reserved.Legal|Privacy policy|Modern Slavery Act Transparency Statement|Sitemap