Fabric defect detection method based on deep separable convolutional neural network
A technology of convolutional neural network and detection method, which is applied in the field of fabric defect detection based on depth separable convolutional neural network, can solve the problems of reduced network calculation amount, large model calculation amount, slow detection speed, etc., so as to improve the detection ability. , High detection accuracy, the effect of improving detection speed
- Summary
- Abstract
- Description
- Claims
- Application Information
AI Technical Summary
Problems solved by technology
Method used
Image
Examples
Embodiment Construction
[0046] The following will clearly and completely describe the technical solutions in the embodiments of the present invention with reference to the accompanying drawings in the embodiments of the present invention. Obviously, the described embodiments are only some, not all, embodiments of the present invention. Based on the embodiments of the present invention, all other embodiments obtained by persons of ordinary skill in the art without making creative efforts belong to the protection scope of the present invention.
[0047] Such as figure 1 As shown, the embodiment of the present invention provides a fabric defect detection method based on a deep separable convolutional neural network, and the specific steps are as follows:
[0048] S1. Use the image acquisition system to collect images of fabric defects and perform preprocessing. The image acquisition system includes four high-definition industrial cameras, which collect 500 high-definition fabric defect images with a res...
PUM
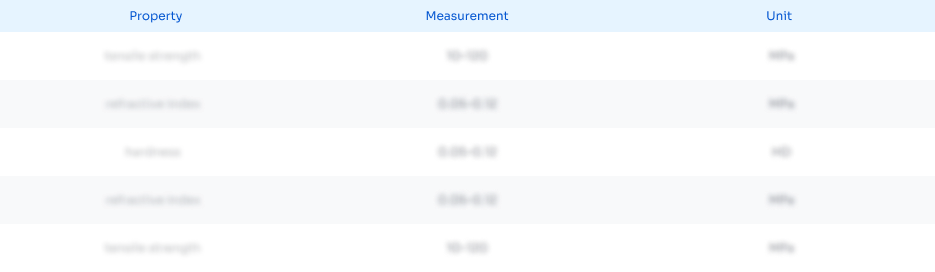
Abstract
Description
Claims
Application Information
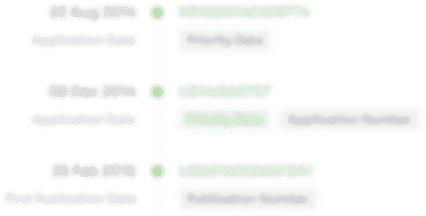
- R&D Engineer
- R&D Manager
- IP Professional
- Industry Leading Data Capabilities
- Powerful AI technology
- Patent DNA Extraction
Browse by: Latest US Patents, China's latest patents, Technical Efficacy Thesaurus, Application Domain, Technology Topic.
© 2024 PatSnap. All rights reserved.Legal|Privacy policy|Modern Slavery Act Transparency Statement|Sitemap