Graph-convolutional-neural-network-based auxiliary diagnosis method for Alzheimer's disease
A convolutional neural network and Alzheimer's disease technology, applied in diagnosis, diagnostic recording/measurement, medical science, etc., can solve problems such as unsatisfactory model classification performance and difficulty in further mining brain neuroimaging data , to achieve accurate classification results and abnormal nodes, eliminating the effect of feature selection steps
- Summary
- Abstract
- Description
- Claims
- Application Information
AI Technical Summary
Problems solved by technology
Method used
Image
Examples
Embodiment Construction
[0028] The invention provides an auxiliary diagnosis method for Alzheimer's disease based on a graph convolutional neural network, which is a fully automatic computer-aided detection method for Alzheimer's disease based on functional magnetic resonance images of the brain. The deep learning method can analyze the functional magnetic resonance images of the brain, and use the graph convolutional neural network to classify and recognize the extracted features, so as to detect the disease state. Compared with the traditional machine learning method, the detection accuracy is significantly improved. . The method model automatically learns the strategy of feature selection and feature fusion, which is completely driven by data, and uses a graph convolutional neural network to train to obtain a model for classification and diagnosis. Compared with the traditional method, the method of the present invention utilizes the advantages of deep learning to automatically learn features and ...
PUM
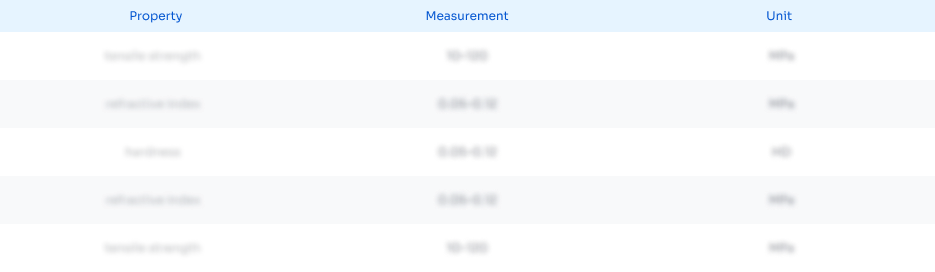
Abstract
Description
Claims
Application Information
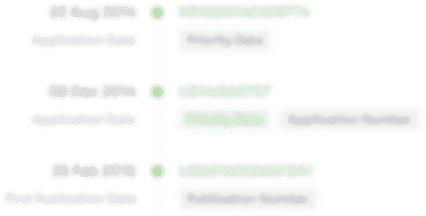
- R&D Engineer
- R&D Manager
- IP Professional
- Industry Leading Data Capabilities
- Powerful AI technology
- Patent DNA Extraction
Browse by: Latest US Patents, China's latest patents, Technical Efficacy Thesaurus, Application Domain, Technology Topic.
© 2024 PatSnap. All rights reserved.Legal|Privacy policy|Modern Slavery Act Transparency Statement|Sitemap