PCB defect detection method based on semantic segmentation
A PCB board and semantic segmentation technology, applied in the direction of optical testing flaws/defects, material analysis, image analysis, etc., can solve the problems of large manpower consumption, low detection efficiency, manual misjudgment, etc., achieve high-precision defect detection and reduce labor Effect of intervention, batch detection
- Summary
- Abstract
- Description
- Claims
- Application Information
AI Technical Summary
Problems solved by technology
Method used
Image
Examples
Embodiment 1
[0053] Such as figure 1 , figure 2 with image 3 As shown, the present embodiment discloses a method for detecting defects of PCB boards based on semantic segmentation, and the method for detecting defects mainly includes the following specific steps:
[0054] Step S1: Data collection and defect definition.
[0055] Specifically, the step S1 specifically includes: collecting from the factory production site a large number of PCB board pictures whose defects cannot be judged under the detection device under the traditional automated process flow and a corresponding number of template diagrams, defining the template diagram as its PCB board to be detected defects standard.
[0056] Specifically, the step S1 further includes: determining the defect type of the PCB board in combination with the requirements of the manufacturer and the knowledge of industry experts, and using semantic segmentation and marking software as an image defect marking tool for data labeling.
[0057]...
Embodiment 2
[0095] to combine Figure 1 to Figure 3 As shown, the present embodiment discloses a method for detecting defects of PCB boards based on semantic segmentation, which is characterized in that it specifically includes the following implementation steps:
[0096] Step 1: Image data acquisition and defect type definition.
[0097] Step 1.1: Collect a large number of PCB board images, unify the collected images into a size of 572*572 (length*width), and prepare the corresponding template image for each image;
[0098] Step 1.2: Define PCB board defect type standards based on manufacturer's needs and industry knowledge. The defect types include: open circuit, short circuit, hole slope, burr, gap, copper slag, thin line, and pinhole.
[0099] Step 2: Image data cleaning and labeling.
[0100] Step 2.1: Clean the image data collected in step 1, use semantic segmentation and labeling software to mark defect features, and generate a json file for storage after marking;
[0101] Step ...
PUM
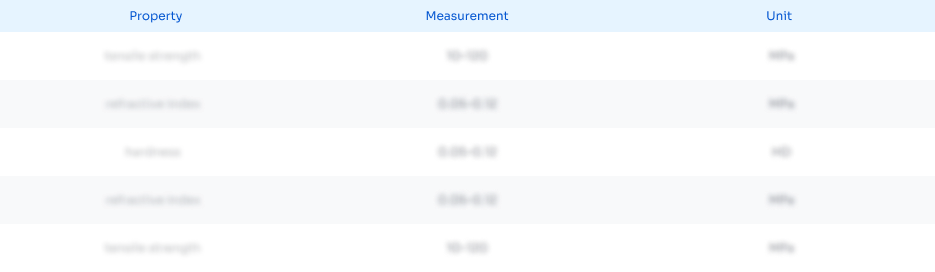
Abstract
Description
Claims
Application Information
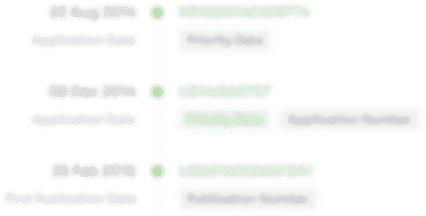
- R&D Engineer
- R&D Manager
- IP Professional
- Industry Leading Data Capabilities
- Powerful AI technology
- Patent DNA Extraction
Browse by: Latest US Patents, China's latest patents, Technical Efficacy Thesaurus, Application Domain, Technology Topic, Popular Technical Reports.
© 2024 PatSnap. All rights reserved.Legal|Privacy policy|Modern Slavery Act Transparency Statement|Sitemap|About US| Contact US: help@patsnap.com