Multi-factor short-term load prediction method based on PCA-DBILSTM
A short-term load forecasting and multi-factor technology, applied in forecasting, neural learning methods, biological neural network models, etc., can solve the problems of inability to process sequence data, limited RNN storage capacity, and high calculation time
- Summary
- Abstract
- Description
- Claims
- Application Information
AI Technical Summary
Problems solved by technology
Method used
Image
Examples
Embodiment 1
[0067] 1. Extraction of load influencing factors
[0068] Since the power load is often affected by many factors such as economy, climate, and holidays, it needs to be considered in the load forecasting model. In order to reduce the input dimension of the neural network and improve the computational efficiency without affecting the prediction accuracy. The present invention uses the PCA method to preprocess the original load data.
[0069] The principle of PCA is to combine high-dimensional historical data into a matrix and perform a series of linear transformations to obtain several uncorrelated linear combinations, so that the new linear combination can reflect the original information as much as possible under the premise of being uncorrelated. . If there is an n-dimensional original data, the k (k<n)-dimensional orthogonalized feature vector is obtained after PCA processing, and we call this k-dimensional orthogonalized feature the principal component. (For the PCA meth...
PUM
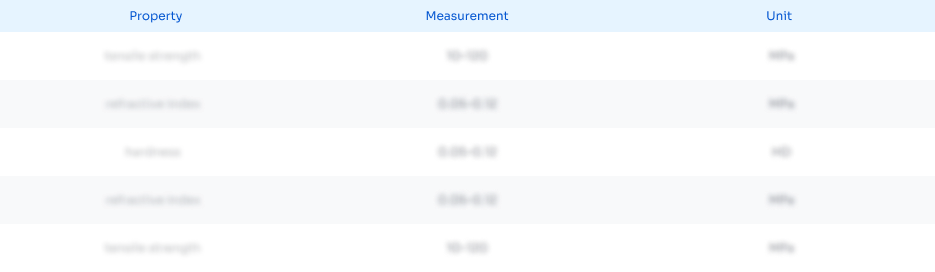
Abstract
Description
Claims
Application Information
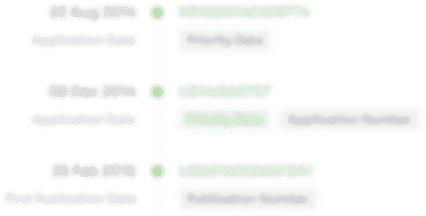
- Generate Ideas
- Intellectual Property
- Life Sciences
- Materials
- Tech Scout
- Unparalleled Data Quality
- Higher Quality Content
- 60% Fewer Hallucinations
Browse by: Latest US Patents, China's latest patents, Technical Efficacy Thesaurus, Application Domain, Technology Topic, Popular Technical Reports.
© 2025 PatSnap. All rights reserved.Legal|Privacy policy|Modern Slavery Act Transparency Statement|Sitemap|About US| Contact US: help@patsnap.com