Adversarial example defense method based on two-stage adversarial knowledge migration
A knowledge and example technology, applied in the field of adversarial example defense based on two-stage confrontational knowledge transfer, can solve the problem of inability to improve the defense ability of simple DNN against adversarial examples, poor effect, difficult training classification accuracy and robustness, etc. question
- Summary
- Abstract
- Description
- Claims
- Application Information
AI Technical Summary
Problems solved by technology
Method used
Image
Examples
Embodiment 1
[0070] 1. Background knowledge
[0071] 1.1 Adversarial examples and threat models
[0072] Adversarial examples widely exist in data such as images, speech, and text. Taking the image classification system as an example, the image adversarial example is a kind of unnatural image based on the natural image, which can be cleverly designed to deceive the deep neural network through careful design. The present invention gives a formal definition of an adversarial example:
[0073] Adversarial example: Let x be a normal data sample, y true is the correct classification label of x, f(·) is a machine learning classifier, and F(·) is a human perceptual judgment. There is a disturbance δ such that f(x+δ)≠y true , and F(x+δ)=y true , then the present invention calls x′=x+δ an adversarial example.
[0074] The present invention refers to such an attack of deceiving a classifier by using an adversarial example as an adversarial attack. The essence of adversarial attacks is to find...
PUM
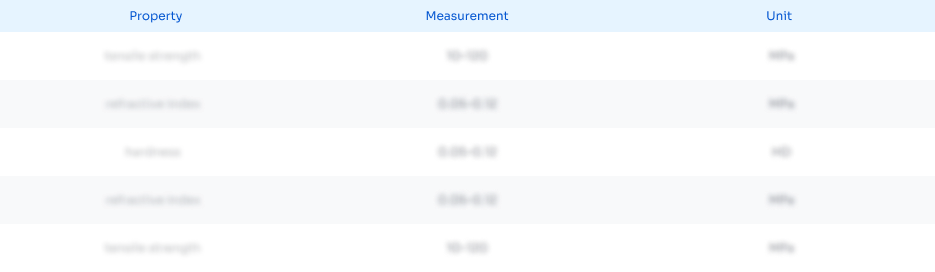
Abstract
Description
Claims
Application Information
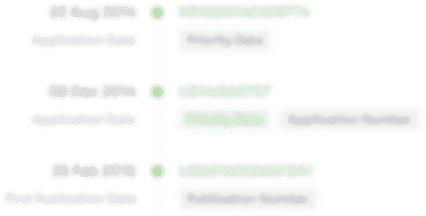
- Generate Ideas
- Intellectual Property
- Life Sciences
- Materials
- Tech Scout
- Unparalleled Data Quality
- Higher Quality Content
- 60% Fewer Hallucinations
Browse by: Latest US Patents, China's latest patents, Technical Efficacy Thesaurus, Application Domain, Technology Topic, Popular Technical Reports.
© 2025 PatSnap. All rights reserved.Legal|Privacy policy|Modern Slavery Act Transparency Statement|Sitemap|About US| Contact US: help@patsnap.com