Image recognition method for breaking faults of wagon brake beam strut
A railway freight car and image recognition technology, which is applied in image enhancement, image analysis, image data processing, etc., can solve the problems of low detection accuracy and poor stability, and achieve the effect of unified operation standards, high accuracy, and fast speed
- Summary
- Abstract
- Description
- Claims
- Application Information
AI Technical Summary
Problems solved by technology
Method used
Image
Examples
specific Embodiment approach 1
[0029] Specific implementation mode 1: The specific process of the fault image recognition method for the breakage of the brake beam pillar of the railway freight car in this implementation mode is as follows:
[0030] Step 1. Create a sample data set;
[0031] Step 2. Based on the sample data set, find the optimal weight coefficient and obtain the trained SSD deep learning network; the specific process is:
[0032] Step 21: Initialize the weight coefficients in a random manner;
[0033] Step 2 and 2: Extract multi-scale features from the sample data set to increase the receptive field; SSD is a multi-scale feature map detection network structure, which extracts feature maps of different scales for detection, large-scale feature maps (the earlier feature map) can be used to detect small objects, while small-scale feature maps (later feature maps) are used to detect large objects. The receptive fields included in the feature map selected by the model are: 38, 19, 10, 5, 3, 1....
specific Embodiment approach 2
[0040] Embodiment 2: The difference between this embodiment and Embodiment 1 is that the sample data set is established in the step 1; the specific process is:
[0041] Set up high-definition equipment around the truck track respectively. After the truck passes through the equipment, obtain all-round high-definition images of the sides, bottom and upper part of the truck; the image is a clear grayscale image; because the parts of the truck may be affected by rain, mud, oil , black paint, foreign matter, ice and snow, chalk writing and other natural or man-made conditions. Also, there may be differences in the images taken by different sites. Therefore, brake beam strut images vary wildly from one to another. Therefore, in the process of collecting the image data of the brake beam pillar, it is necessary to ensure the diversity, and try to collect all the images of the brake beam pillar under various conditions.
[0042] In freight car bogies of different models, the shape of...
specific Embodiment approach 3
[0053] Specific embodiment three: the difference between this embodiment and specific embodiment one or two is that the default frame (anchor box) is generated in the step two or three; the specific process is:
[0054] For each feature map, k default boxes (default boxes [default box]) are generated according to different sizes (scale) and aspect ratios (ratio);
[0055] The formula for each default box size is:
[0056] Among them, m is the number of feature maps, s min is the size of the feature map at the bottom layer, s max Default box size for the topmost feature map;
[0057] The aspect ratio of each default box is calculated according to the ratio value, and the ratio value is {1,2,3,1 / 2,1 / 3};
[0058] For the default box with a scale of 1, add an additional scale of the default box;
[0059] In the formula, s' k For the increased ratio ({1,2,3,1 / 2,1 / 3}, there are a total of 5 silent boxes (one number represents one), this is the size of the increased default bo...
PUM
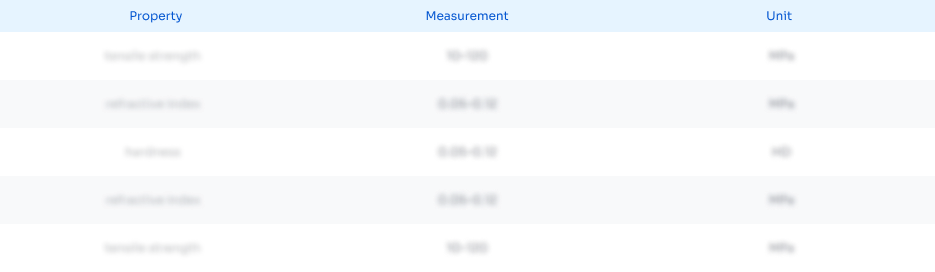
Abstract
Description
Claims
Application Information
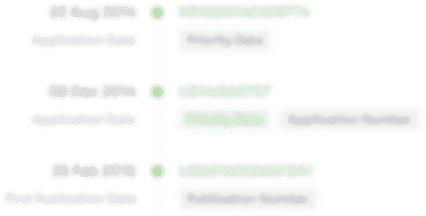
- R&D Engineer
- R&D Manager
- IP Professional
- Industry Leading Data Capabilities
- Powerful AI technology
- Patent DNA Extraction
Browse by: Latest US Patents, China's latest patents, Technical Efficacy Thesaurus, Application Domain, Technology Topic.
© 2024 PatSnap. All rights reserved.Legal|Privacy policy|Modern Slavery Act Transparency Statement|Sitemap