Multi-scale image segmentation method based on weight learning
An image segmentation, multi-scale technology, applied in the field of remote sensing image processing, can solve a lot of time, labor and other problems, and achieve the effect of high accuracy and recall rate
- Summary
- Abstract
- Description
- Claims
- Application Information
AI Technical Summary
Problems solved by technology
Method used
Image
Examples
Embodiment Construction
[0032] The specific implementation of the method of the present invention will be further described below in conjunction with the accompanying drawings.
[0033] Such as figure 1 As shown, a multi-scale image convolutional layer feature learning method based on weight learning includes the following steps:
[0034] 1) put the sample into the model designed by the present invention for training;
[0035] The structure of the model designed by the present invention is: encoding-decoding structure
[0036] 2) Input the remote sensing image of the test area as the input source into the model in 1);
[0037] 3) Use the encoder to encode the features of the image of the test area to obtain five pooled features of different scales. The encoder uses five downsampling modules, the first two downsampling modules contain two convolutional modules plus one pooling layer, the last three downsampling modules contain three convolutional modules and one pooling layer, and the convolutional...
PUM
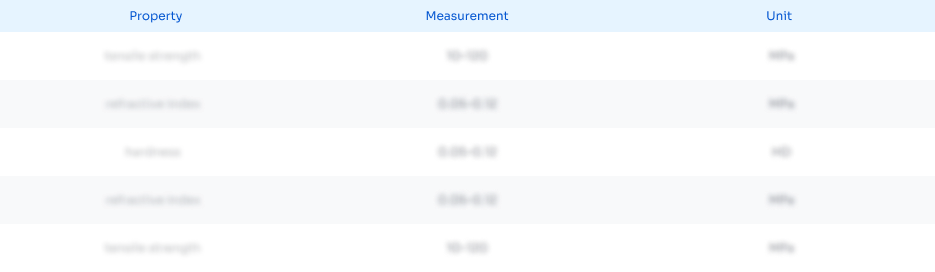
Abstract
Description
Claims
Application Information
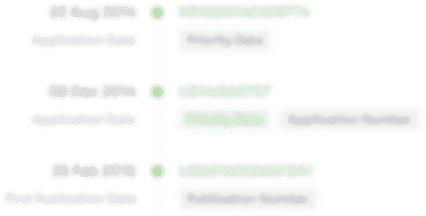
- R&D Engineer
- R&D Manager
- IP Professional
- Industry Leading Data Capabilities
- Powerful AI technology
- Patent DNA Extraction
Browse by: Latest US Patents, China's latest patents, Technical Efficacy Thesaurus, Application Domain, Technology Topic, Popular Technical Reports.
© 2024 PatSnap. All rights reserved.Legal|Privacy policy|Modern Slavery Act Transparency Statement|Sitemap|About US| Contact US: help@patsnap.com