Digestive tract disease auxiliary diagnosis system based on deep learning
A technology for gastrointestinal diseases and auxiliary diagnosis, applied in the field of artificial intelligence, can solve the problems of low accuracy of human eye diagnosis, slow manual inspection, and large number of biopsies, so as to improve the efficiency of auxiliary diagnosis and treatment and scientific research, and improve the level of screening. , the effect of reducing the burden on doctors
- Summary
- Abstract
- Description
- Claims
- Application Information
AI Technical Summary
Problems solved by technology
Method used
Image
Examples
Embodiment approach
[0073] As another implementation, the lesion area positioning module further includes:
[0074] Digestive tract lesion area recognition model training module, which is used to acquire multiple images of digestive tract parts containing known digestive tract lesions, such as Figure 3(a)-Figure 3(d) , and label the known gastrointestinal lesion area, the labeling results are as follows Figure 4 As shown; specify the existing network model architecture, or build a custom network module architecture; receive model training parameters, and train the digestive tract lesion area recognition model according to the labeled training images. Identify the digestive tract lesion area and the final output labeling results, such as Figure 5 shown.
[0075] As an implementation, the existing network model architecture adopts the YOLO v3 neural network.
[0076] Utilizing its characteristics of high detection accuracy and fast detection speed, it can meet the needs of electronic gastrosc...
PUM
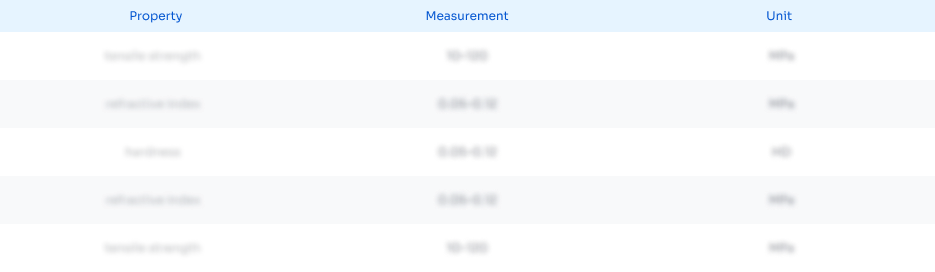
Abstract
Description
Claims
Application Information
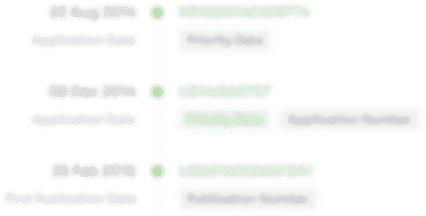
- R&D Engineer
- R&D Manager
- IP Professional
- Industry Leading Data Capabilities
- Powerful AI technology
- Patent DNA Extraction
Browse by: Latest US Patents, China's latest patents, Technical Efficacy Thesaurus, Application Domain, Technology Topic.
© 2024 PatSnap. All rights reserved.Legal|Privacy policy|Modern Slavery Act Transparency Statement|Sitemap