Hyperspectral image unmixing method based on multi-layer stacked autoencoder
An auto-encoder, hyperspectral image technology, applied in instruments, character and pattern recognition, biological neural network models, etc., can solve problems affecting abundance estimation, low unmixing accuracy, difficult practical application, etc., and achieve generalization ability Strong, improve the effect of unmixing accuracy
- Summary
- Abstract
- Description
- Claims
- Application Information
AI Technical Summary
Problems solved by technology
Method used
Image
Examples
Embodiment Construction
[0041] In order to deepen the understanding of the present invention, the present invention will be further described below in conjunction with the examples, which are only used to explain the present invention, and do not constitute a limitation to the protection scope of the present invention.
[0042] according to figure 1 , 2 , 3, the present embodiment proposes a hyperspectral image unmixing method based on a multi-layer stacked autoencoder, including the following steps:
[0043] Step 1: Train three autoencoders one by one to form a stacked autoencoder, extract high-order spectral features of the image, and obtain feature layer 1, feature layer 2, and feature layer 3. The three autoencoders are regarded as one in terms of network structure. A feed-forward neural network consists of an input layer, one or more feature layers and an output layer, expressed mathematically as: input data x∈R m*n, mapping it to the feature layer a ∈ R m*h , then a is decoded to the output ...
PUM
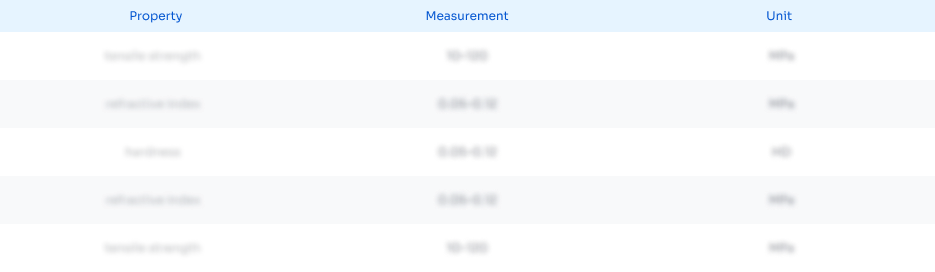
Abstract
Description
Claims
Application Information
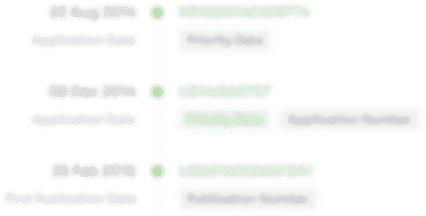
- R&D Engineer
- R&D Manager
- IP Professional
- Industry Leading Data Capabilities
- Powerful AI technology
- Patent DNA Extraction
Browse by: Latest US Patents, China's latest patents, Technical Efficacy Thesaurus, Application Domain, Technology Topic, Popular Technical Reports.
© 2024 PatSnap. All rights reserved.Legal|Privacy policy|Modern Slavery Act Transparency Statement|Sitemap|About US| Contact US: help@patsnap.com