A level set and deep learning combined retinal layer segmentation method and system
A technology of deep learning network and retinal layer, which is applied in the field of OCT retinal layer segmentation method and system, can solve a large number of labeled samples and other problems, and achieve the effect of saving computation and fine segmentation
- Summary
- Abstract
- Description
- Claims
- Application Information
AI Technical Summary
Problems solved by technology
Method used
Image
Examples
Embodiment 1
[0055] This embodiment provides an OCT retinal layer segmentation method based on the combination of the level set model and the FCN deep learning network. The method preprocesses the images collected by the optical coherence tomography equipment, and then uses the prior information of the retinal layer thickness , evolve the active contour to the boundary to be segmented through the level set model.
[0056] Please refer to the attached figure 1 , the OCT retinal layer segmentation method comprises the following steps:
[0057] S101. Collect normal retinal OCT images and diseased retinal OCT images, and perform preprocessing on the normal retinal OCT images and diseased retinal OCT images respectively.
[0058] Specifically, optical coherence tomography (OCT) equipment is used to collect normal retinal OCT images and lesioned retinal layer OCT images respectively, and de-drying and other preprocessing are performed on the normal retinal OCT images and lesioned retinal OCT im...
Embodiment 2
[0088] Please refer to the attached figure 2 , this embodiment provides a retinal layer segmentation system combining level set and deep learning, the system includes:
[0089] The image acquisition module is used to collect normal retinal OCT images and diseased retinal OCT images, and make classification labels;
[0090] The data set construction module is used to process normal retinal OCT images and diseased retinal OCT images, and construct training sets and test sets;
[0091] A model training module for training a deep learning network using the image data in the training set;
[0092] The rough segmentation module is used to process the image data in the test set by using the trained deep learning network to obtain the rough classification result of the retinal layer;
[0093] The contour evolution module is used to use the result of the rough classification of the retinal layer as a level set function, and use the gradient descent method to evolve each level set fu...
PUM
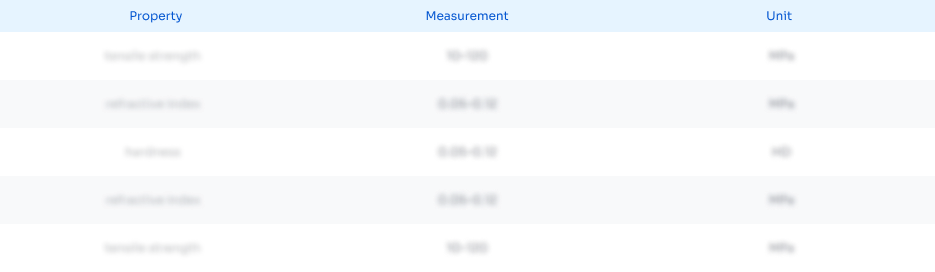
Abstract
Description
Claims
Application Information
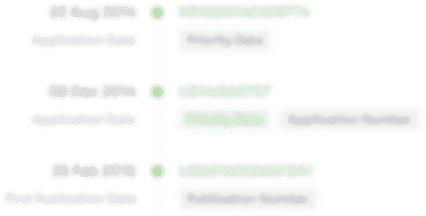
- R&D Engineer
- R&D Manager
- IP Professional
- Industry Leading Data Capabilities
- Powerful AI technology
- Patent DNA Extraction
Browse by: Latest US Patents, China's latest patents, Technical Efficacy Thesaurus, Application Domain, Technology Topic, Popular Technical Reports.
© 2024 PatSnap. All rights reserved.Legal|Privacy policy|Modern Slavery Act Transparency Statement|Sitemap|About US| Contact US: help@patsnap.com