Short-term wind speed prediction method integrating deep learning and data denoising
A technology of wind speed forecasting and deep learning, applied in forecasting, data processing applications, electrical digital data processing, etc., can solve problems such as difficult to use, affecting the initial decomposition subsequence value, and reducing accuracy
- Summary
- Abstract
- Description
- Claims
- Application Information
AI Technical Summary
Problems solved by technology
Method used
Image
Examples
Embodiment 1
[0063] see Figure 1 to Figure 3 , a short-term wind speed prediction method integrating deep learning and data denoising, mainly includes the following steps:
[0064] 1) Obtain the original wind speed sequence, and preprocess the original wind speed sequence to obtain the wind speed sequence A:{α 1 ,α 2 ,…,α l}. l is the length of the data sequence. The preprocessing includes linear interpolation and normalization. alpha l is the wind speed.
[0065] 2) Use discrete wavelet transform to decompose the wind speed sequence A to obtain the proportional coefficient c containing low-frequency information m,n and wavelet coefficients d containing high-frequency information m,n .
[0066] The main steps of decomposing the wind speed sequence A using discrete wavelet transform are as follows:
[0067] 2.1) Set the wavelet function as a binary wavelet, that is, let a=2 m ,b=n2 m ,m,n∈Z. a is a scale factor, b is a time shift factor, m is a scale, and n is a parameter rela...
Embodiment 2
[0116] An experiment to verify a short-term wind speed prediction method integrating deep learning and data denoising mainly includes the following steps:
[0117] 1) Obtain the original wind speed sequence; perform linear interpolation of missing values and data normalization on the collected original wind speed sequence through data preprocessing, and obtain the sorted wind speed sequence A:{α 1 ,α 2 ,…,α l} (l is the length of the data sequence).
[0118] Since there are many missing and erroneous values in the wind speed raw data, the data is preprocessed using a linear interpolation technique: first, missing and erroneous points are replaced with a flag called "Not a Number (NaN)". Then, the NaN positions are patched using a linear interpolation method. Similarly, convert a raw data series with a resolution of minutes to data with a resolution of hours. Additionally, these time series are normalized to the interval -1 to 1.
[0119] 2) Use discrete wavelet transf...
PUM
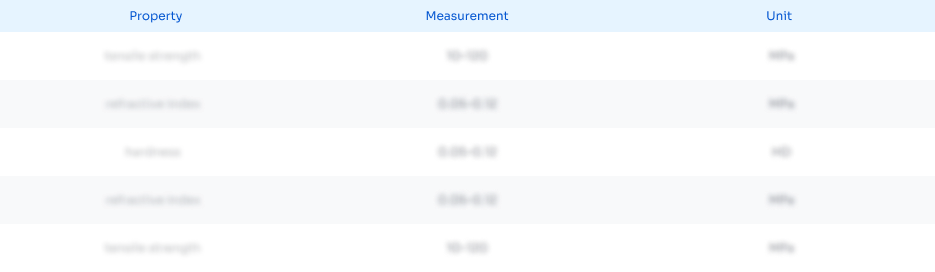
Abstract
Description
Claims
Application Information
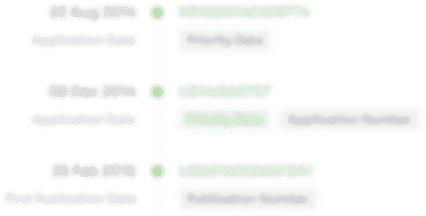
- R&D Engineer
- R&D Manager
- IP Professional
- Industry Leading Data Capabilities
- Powerful AI technology
- Patent DNA Extraction
Browse by: Latest US Patents, China's latest patents, Technical Efficacy Thesaurus, Application Domain, Technology Topic, Popular Technical Reports.
© 2024 PatSnap. All rights reserved.Legal|Privacy policy|Modern Slavery Act Transparency Statement|Sitemap|About US| Contact US: help@patsnap.com