Point cloud classification method based on multi-level aggregation feature extraction and fusion
A technology of feature extraction and classification method, applied in the direction of instruments, character and pattern recognition, computer parts, etc., can solve the problem of lack of local structure of point set, and achieve the effect of robust point set feature
- Summary
- Abstract
- Description
- Claims
- Application Information
AI Technical Summary
Problems solved by technology
Method used
Image
Examples
Embodiment Construction
[0018] like figure 1 shown. First, for the input point cloud data, a multi-level point set construction method based on point cloud density and point set maximum point control is used to generate a multi-layer point set. Then, extract multi-scale covariance eigenvalue features and Spin Image features for each single point of the point cloud. Then, LLC is used for dictionary learning and sparse representation on the single-point features of the point cloud. Combined with the sparse representation of multi-level point sets and single points, the LDA model and multi-scale maximum pooling method are used to generate global point set LLC-LDA features and local point set LLC-MP features. Then, the different types of point set features at different levels are transferred to the finest layer point set space, and then the multi-level aggregation features of point sets are constructed, and different types of features are fused. Finally, based on the multi-level aggregation feature of...
PUM
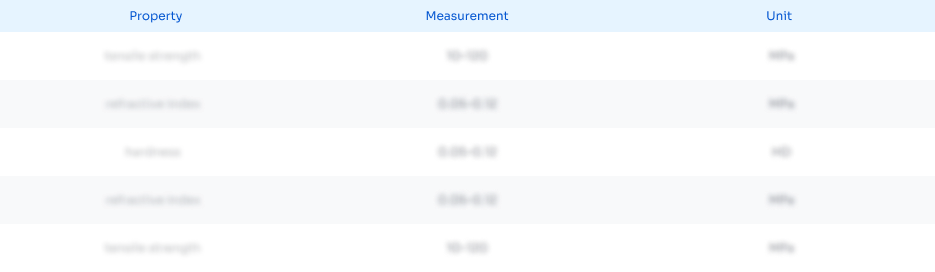
Abstract
Description
Claims
Application Information
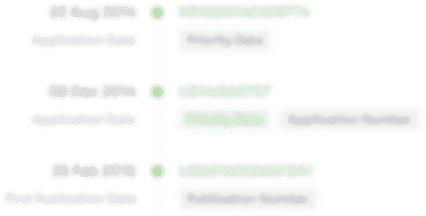
- R&D Engineer
- R&D Manager
- IP Professional
- Industry Leading Data Capabilities
- Powerful AI technology
- Patent DNA Extraction
Browse by: Latest US Patents, China's latest patents, Technical Efficacy Thesaurus, Application Domain, Technology Topic, Popular Technical Reports.
© 2024 PatSnap. All rights reserved.Legal|Privacy policy|Modern Slavery Act Transparency Statement|Sitemap|About US| Contact US: help@patsnap.com