Tourism named entity identification method based on BBLC model
A named entity recognition and model technology, applied in the field of semantic recognition, can solve the problems of lack of feature marks in Chinese words, inability to distinguish the ambiguity of the same word, difficulty in named entity recognition, etc., and achieve high F value, good effect, and strong generalization ability. Effect
- Summary
- Abstract
- Description
- Claims
- Application Information
AI Technical Summary
Problems solved by technology
Method used
Image
Examples
Embodiment
[0061] This embodiment uses BIO notation to mark entities. BIO rules are: B represents the beginning of an entity, I represents the middle entity and O represents other non-entity words.
[0062] The authoritative SIGHAN 2006 off-3 corpus only contains the annotations of three entities, PER, LOC and ORG respectively represent the name of the person, the name of the location and the name of the organization; it will take time to fully characterize the various entities of the tourism data , time and other marked entities, so this paper chooses to crawl travel notes, strategies, comments and other text data of travel websites such as Ctrip and Mafengwo. A total of 13464 Chinese sentences are used as experimental data after BIO marking of 15431 entities in five categories in the text The corresponding sets, labels and entities are shown in Table 1:
[0063] Table 1 Entity label set
[0064]
PUM
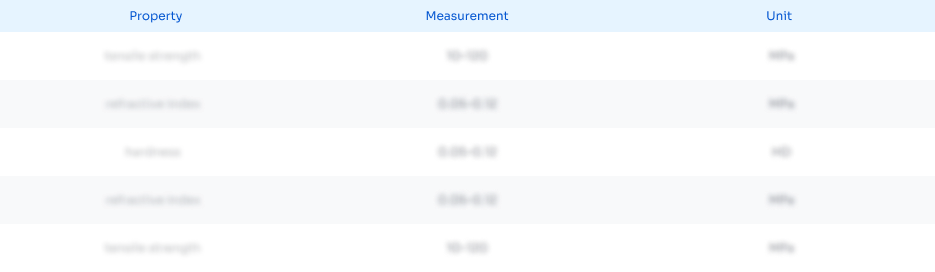
Abstract
Description
Claims
Application Information
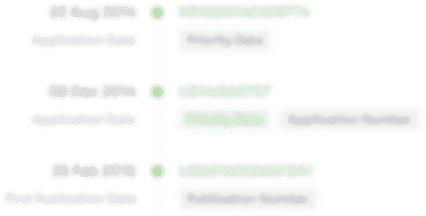
- R&D Engineer
- R&D Manager
- IP Professional
- Industry Leading Data Capabilities
- Powerful AI technology
- Patent DNA Extraction
Browse by: Latest US Patents, China's latest patents, Technical Efficacy Thesaurus, Application Domain, Technology Topic, Popular Technical Reports.
© 2024 PatSnap. All rights reserved.Legal|Privacy policy|Modern Slavery Act Transparency Statement|Sitemap|About US| Contact US: help@patsnap.com