Attack defense training method based on generative adversarial network
A training method and network technology, applied in biological neural network models, neural learning methods, instruments, etc., can solve problems such as the difference between the network boundary and the real decision boundary, modifying data and using additional networks to increase workload, etc.
- Summary
- Abstract
- Description
- Claims
- Application Information
AI Technical Summary
Problems solved by technology
Method used
Image
Examples
Embodiment 1
[0085] figure 1 A flowchart showing a method for training defense against adversarial attacks based on generative adversarial networks, figure 2 Represents the training framework of adversarial attack defense based on generative adversarial networks, including generator G, discriminator D and target network F, attack algorithm library Ω attack .
[0086] Among them, in this example, the generator G uses the basic residual module of ResNet as a deconvolutional neural network to upsample tensors, random noise z and random condition vector c fake As the input of the generator G, the fake sample image x is obtained after deconvolution network upsampling fake ; The discriminator D uses ResNet as the network structure and receives the attack algorithm library Ω attack Taking the target network F as the attack target, the attack sample after processing and generate samples x fakeThe target network F uses VGG as the network structure, and finally retains the parameters of the d...
PUM
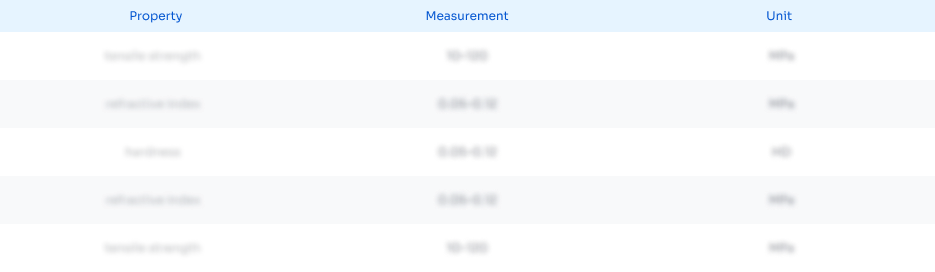
Abstract
Description
Claims
Application Information
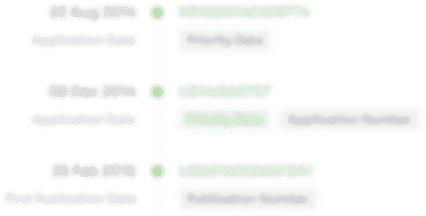
- R&D Engineer
- R&D Manager
- IP Professional
- Industry Leading Data Capabilities
- Powerful AI technology
- Patent DNA Extraction
Browse by: Latest US Patents, China's latest patents, Technical Efficacy Thesaurus, Application Domain, Technology Topic, Popular Technical Reports.
© 2024 PatSnap. All rights reserved.Legal|Privacy policy|Modern Slavery Act Transparency Statement|Sitemap|About US| Contact US: help@patsnap.com