Infrared thermal image nondestructive testing method based on convolutional neural network
A technology of convolutional neural network and infrared thermal imaging, which is applied in the field of non-destructive testing of infrared thermal imaging, to achieve the effect of large detection range, intuitive detection range and accurate detection
- Summary
- Abstract
- Description
- Claims
- Application Information
AI Technical Summary
Problems solved by technology
Method used
Image
Examples
specific Embodiment approach 1
[0035] A nondestructive detection method for infrared thermal images based on a convolutional neural network in this embodiment, such as figure 1 As shown, the method is realized through the following steps:
[0036] Step 1. Arrange the infrared image data collection scene, and collect the defective infrared image of the object to be tested;
[0037] Step 2, performing enhancement and noise reduction processing on the collected defective infrared image of the object to be measured, and completing the preprocessing process;
[0038] Step 3, using the preprocessed infrared images with defects of the object to be tested to augment and construct the data set;
[0039] Step 4, merging the models of VGG16 and DenseNet169 networks, and using the data set in step 3 to train and test the fused model;
[0040] Step 5, using the fused network model to identify and detect defects in the defective infrared image of the object to be tested.
specific Embodiment approach 2
[0042] The difference from the first specific embodiment is that in this embodiment, a non-destructive detection method for infrared thermal images based on convolutional neural network, in the second step, the collected infrared images with defects of the object under test are enhanced and reduced. Noise processing, complete the preprocessing process, specifically:
[0043] First, calculate the multi-contrast joint image of absolute contrast, change contrast, normalized contrast, standard contrast and differential absolute contrast for the collected infrared image, so as to enhance the defect part in the infrared image;
[0044] In addition, the wavelet transform threshold is used to remove noise, and the non-uniform heating, noise and distortion existing in the image are removed. Since the infrared imaging system is susceptible to interference, and there will be uneven heating in the image, compared with the visible light image, the noise is greater. Infrared detection itsel...
specific Embodiment approach 3
[0047] The difference from the second embodiment is that in this embodiment, a non-destructive detection method for infrared thermal images based on convolutional neural network, the use of wavelet transform threshold to remove noise, remove uneven heating, noise and distortion existing in the image The process is:
[0048] First, decompose the red signal;
[0049] Then, according to the energy concentration of the signal after the wavelet transform, that is, the characteristic that it usually concentrates on the larger wavelet coefficients, if you want to obtain the signal, you must separately process the wavelet coefficients at high and low resolutions, and retain the large wavelet coefficients. The wavelet coefficients of low-resolution scales; the wavelet coefficients corresponding to high-resolution in other scales cannot be directly determined to be retained. It is necessary to set a threshold, compare the amplitude of these wavelet coefficients with the threshold, and r...
PUM
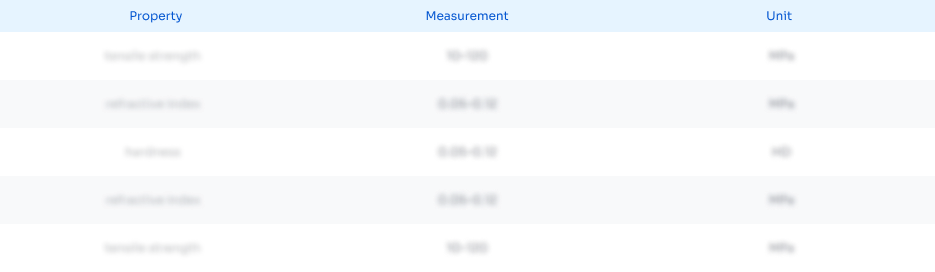
Abstract
Description
Claims
Application Information
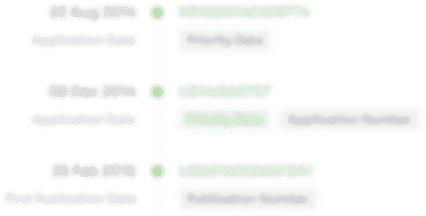
- R&D
- Intellectual Property
- Life Sciences
- Materials
- Tech Scout
- Unparalleled Data Quality
- Higher Quality Content
- 60% Fewer Hallucinations
Browse by: Latest US Patents, China's latest patents, Technical Efficacy Thesaurus, Application Domain, Technology Topic, Popular Technical Reports.
© 2025 PatSnap. All rights reserved.Legal|Privacy policy|Modern Slavery Act Transparency Statement|Sitemap|About US| Contact US: help@patsnap.com