Case-related news viewpoint sentence sentiment classification method based on CNN-BiLSTM + action model
A technology for sentiment classification and opinion sentences, which is applied in the fields of natural language processing and deep learning, can solve complex feature engineering and manual engineering of rule and statistical machine learning methods, and achieve complex feature engineering and redundant manual work and improve classification effects, effects that reduce effort and complexity
- Summary
- Abstract
- Description
- Claims
- Application Information
AI Technical Summary
Problems solved by technology
Method used
Image
Examples
Embodiment
[0052] Example: such as Figure 1-Figure 2 As shown, a method of sentiment classification of news opinion sentences involved in the case based on the CNN-BiLSTM+attention model;
[0053] The CNN-BiLSTM+attention model includes: word embedding layer, convolutional layer, pooling layer, BiLSTM layer, Attention attention layer, Softmax classification layer;
[0054] The word embedding layer is used to convert sentence words into low-dimensional word vectors, the convolutional layer is used to automatically extract word features, the pooling layer is used to reduce the dimension of feature vectors, and the BiLSTM layer is Used to memorize long-term dependent serialized information, the Attention layer is used to reinforce important information with a weight matrix, and the Softmax classification layer is used to classify the emotional category with the highest probability;
[0055] The method includes the following steps:
[0056] Step 1: Preprocess the opinion sentences of the news rela...
PUM
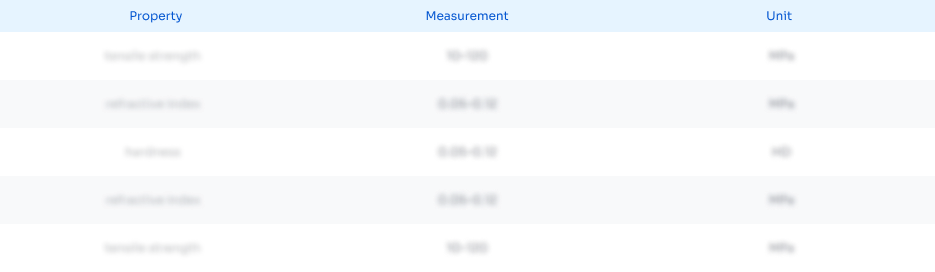
Abstract
Description
Claims
Application Information
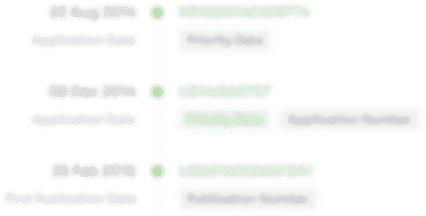
- R&D
- Intellectual Property
- Life Sciences
- Materials
- Tech Scout
- Unparalleled Data Quality
- Higher Quality Content
- 60% Fewer Hallucinations
Browse by: Latest US Patents, China's latest patents, Technical Efficacy Thesaurus, Application Domain, Technology Topic, Popular Technical Reports.
© 2025 PatSnap. All rights reserved.Legal|Privacy policy|Modern Slavery Act Transparency Statement|Sitemap|About US| Contact US: help@patsnap.com