Dynamic safety assessment method based on random forest and extreme learning regression
An extreme learning and dynamic security technology, applied in neural learning methods, biological models, biological neural network models, etc., can solve the problem that the key variables are not enough for classification or regression training, it is difficult to use a large amount of data analysis, and the feature selection is not accurate enough and other problems to achieve the effect of saving offline training time, achieving dimensionality reduction, and accurate regression prediction results.
- Summary
- Abstract
- Description
- Claims
- Application Information
AI Technical Summary
Problems solved by technology
Method used
Image
Examples
Embodiment
[0095] The method proposed by the present invention is tested in the IEEE 39 node example system, and the node system is composed of 10 generators, 39 nodes and 46 lines. This test includes all the steps described in the method of the present invention, and is carried out on a computer equipped with an Intel Core i7 processor and 8GB of memory.
[0096]By collecting historical operation data of the power system from power grid companies and performing simulations based on a series of faults, a total of 4,851 samples were obtained in this test, and the top 0.1% variables of the selected variable importance score were used to construct a sample set. Using the ten-fold sample division method, the sample is roughly divided into ten parts, one part of which is taken as the test sample set each time, and the rest is the training sample set, and the test is repeated ten times until the mean and standard deviation of the accuracy tend to be stable.
[0097] In this test, the residual ...
PUM
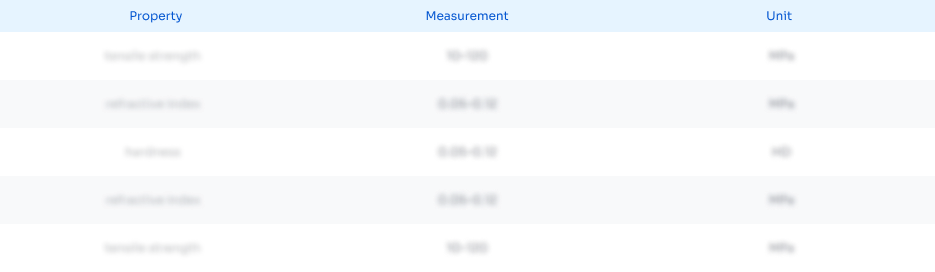
Abstract
Description
Claims
Application Information
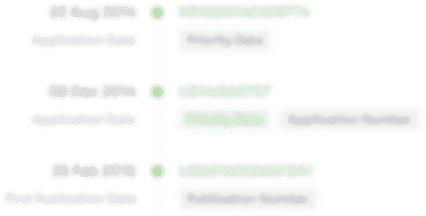
- R&D Engineer
- R&D Manager
- IP Professional
- Industry Leading Data Capabilities
- Powerful AI technology
- Patent DNA Extraction
Browse by: Latest US Patents, China's latest patents, Technical Efficacy Thesaurus, Application Domain, Technology Topic, Popular Technical Reports.
© 2024 PatSnap. All rights reserved.Legal|Privacy policy|Modern Slavery Act Transparency Statement|Sitemap|About US| Contact US: help@patsnap.com