Intrusion detection method for optimizing regularization extreme learning machine through improved longicorn swarm algorithm
An extreme learning machine and intrusion detection technology, which is applied in neural learning methods, calculations, calculation models, etc., can solve problems such as intrusion detection capabilities of algorithms without excellent classification performance, improve detection accuracy and training speed, reduce complexity, and improve The effect of convergence speed
- Summary
- Abstract
- Description
- Claims
- Application Information
AI Technical Summary
Problems solved by technology
Method used
Image
Examples
Embodiment
[0032] Embodiment: a kind of intrusion detection method that improves the Tianniu herd algorithm to optimize the regularized extreme learning machine, such as figure 1 shown, specifically the following steps
[0033] S1: Initialize the parameters of the regularized extreme learning machine model, obtain the input layer nodes, hidden layer nodes and output layer nodes, as well as the initial weight and threshold of the network;
[0034] S2: Obtain the global optimal position by using the improved swarm algorithm, and the optimal position is the optimal initial weight and threshold. Among them, the improved Tianniu algorithm specifically includes the following steps
[0035] S101: Use the Tent mapping reverse learning method to initialize the beetle group, and obtain the input layer nodes, hidden layer nodes and output layer nodes as well as network initial weights and thresholds;
[0036] S102: Calculate the fitness function value of the beetle group according to the training...
PUM
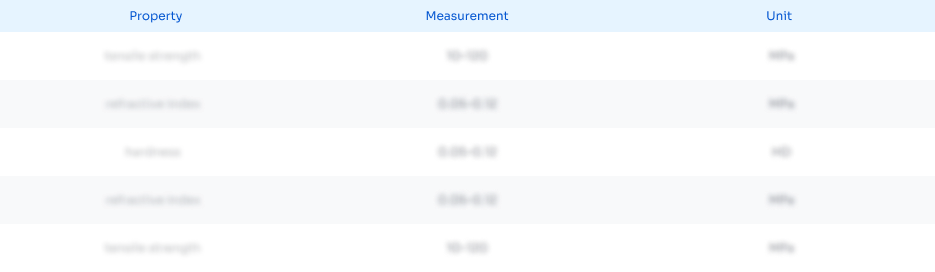
Abstract
Description
Claims
Application Information
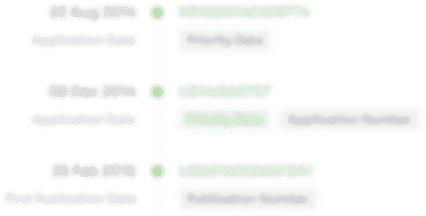
- R&D
- Intellectual Property
- Life Sciences
- Materials
- Tech Scout
- Unparalleled Data Quality
- Higher Quality Content
- 60% Fewer Hallucinations
Browse by: Latest US Patents, China's latest patents, Technical Efficacy Thesaurus, Application Domain, Technology Topic, Popular Technical Reports.
© 2025 PatSnap. All rights reserved.Legal|Privacy policy|Modern Slavery Act Transparency Statement|Sitemap|About US| Contact US: help@patsnap.com