Pipeline 3D reconstruction method, system, medium and equipment based on deep learning
A technology of deep learning and 3D reconstruction, applied in the field of 3D pipeline reconstruction based on deep learning, to achieve good accuracy and convergence, prevent errors, and high robustness
- Summary
- Abstract
- Description
- Claims
- Application Information
AI Technical Summary
Problems solved by technology
Method used
Image
Examples
Embodiment 1
[0043] In piping design, a piping scene is assembled from piping components and piping supports. In this embodiment, due to the complexity of the problem, the pipeline components are mainly considered, and supports such as floors and fences are ignored.
[0044] Choose from six types of components as primitives: pipes, flanges, elbows, reducers, tees, and crosses. Points that do not belong to these six types of components are marked with an additional label. In this embodiment, the classes of components are learned, thus reducing the complexity of the common pipeline reconstruction problem to a combined component detection and model fitting problem.
[0045] In this embodiment, a priori-based learning method is adopted, and a deep learning network is trained to learn candidate features of 3D point clouds. The a priori detection of generating training sets and designing training networks is usually error-prone, so a combination of clustering and graph technology is used to fi...
Embodiment 2
[0129] Embodiment 2 of the present disclosure provides a pipeline three-dimensional reconstruction system based on deep learning, including:
[0130] The point cloud learning module is configured to: use a deep learning method to learn the characteristics of the point cloud, and at least obtain the category of the component to which the point belongs, the radius of the component to which the point belongs, and the direction vector of the point;
[0131] The candidate instance obtaining module is configured to: use the radius of the component to which the point belongs and the direction vector of the point to calculate the axis point, and combine the category labels of the component to which the point belongs to cluster the axis point to obtain a candidate instance;
[0132] The graph structure component module is configured to: use a graph-based method to obtain the connection relationship between different component instances, and use the components as nodes to form a graph st...
Embodiment 3
[0136] Embodiment 3 of the present disclosure provides a medium on which a program is stored, and when the program is executed by a processor, the steps in the deep learning-based pipeline three-dimensional reconstruction method described in Embodiment 1 of the present disclosure are implemented.
PUM
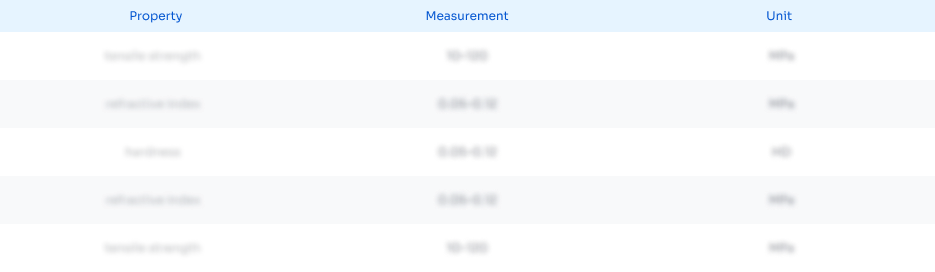
Abstract
Description
Claims
Application Information
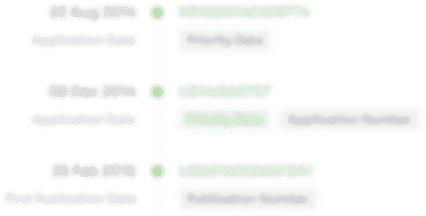
- R&D Engineer
- R&D Manager
- IP Professional
- Industry Leading Data Capabilities
- Powerful AI technology
- Patent DNA Extraction
Browse by: Latest US Patents, China's latest patents, Technical Efficacy Thesaurus, Application Domain, Technology Topic, Popular Technical Reports.
© 2024 PatSnap. All rights reserved.Legal|Privacy policy|Modern Slavery Act Transparency Statement|Sitemap|About US| Contact US: help@patsnap.com