Active suspension reinforcement learning control method based on deep Q neural network
A technology of reinforcement learning and active suspension, applied in the direction of adaptive control, general control system, control/regulation system, etc., can solve complex and nonlinear models, cannot adapt to complex and changeable road conditions, and cannot handle parameter variations well Determination and other issues to achieve the effect of improving ride comfort, good comfort and road adaptability
- Summary
- Abstract
- Description
- Claims
- Application Information
AI Technical Summary
Problems solved by technology
Method used
Image
Examples
Embodiment Construction
[0033] The following will clearly and completely describe the technical solutions in conjunction with the accompanying drawings in the embodiments of the present invention.
[0034] Such as figure 1 As shown, the active suspension reinforcement learning control framework of this embodiment includes the following parts: active suspension reinforcement learning controller body, active suspension system, state observation, active suspension control force and reward. The controller obtains state observations such as the dynamic deflection of the suspension, the acceleration of the vehicle body, and the vertical displacement of the vehicle body from the suspension system, and uses a certain strategy to determine which active force to apply to the suspension in each state. The active force changes the state and generates a reward to judge the quality of the current action; the reinforcement learning algorithm based on the deep Q network is used to update the strategy.
[0035] Such...
PUM
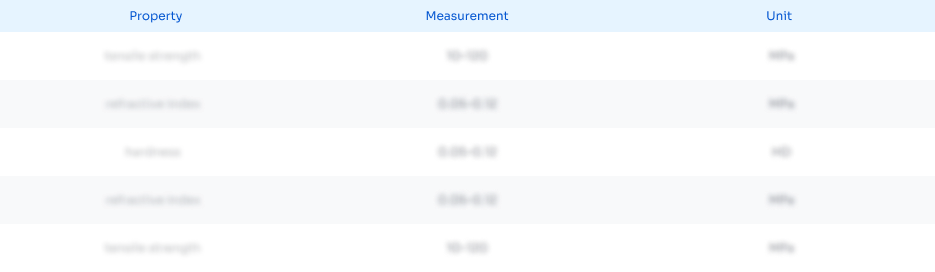
Abstract
Description
Claims
Application Information
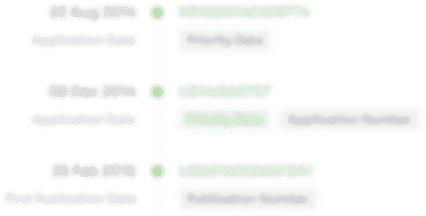
- R&D
- Intellectual Property
- Life Sciences
- Materials
- Tech Scout
- Unparalleled Data Quality
- Higher Quality Content
- 60% Fewer Hallucinations
Browse by: Latest US Patents, China's latest patents, Technical Efficacy Thesaurus, Application Domain, Technology Topic, Popular Technical Reports.
© 2025 PatSnap. All rights reserved.Legal|Privacy policy|Modern Slavery Act Transparency Statement|Sitemap|About US| Contact US: help@patsnap.com