Deep neural network model compression training method, device and apparatus and medium
A technology of deep neural network and training method, which is applied in the direction of biological neural network model, neural learning method, neural architecture, etc., and can solve problems such as limited application, high computational complexity, and reasoning speed that cannot meet real-time requirements
- Summary
- Abstract
- Description
- Claims
- Application Information
AI Technical Summary
Problems solved by technology
Method used
Image
Examples
Embodiment Construction
[0059] The technical solutions in the embodiments of the present application will be clearly and completely described below in conjunction with the accompanying drawings in the embodiments of the present application. Obviously, the described embodiments are only some of the embodiments of the present application, not all of them. Based on the embodiments in this application, all other embodiments obtained by persons of ordinary skill in the art without making creative efforts belong to the scope of protection of this application.
[0060] see figure 1 As shown, the embodiment of the present application discloses a deep neural network model compression training method, the method comprising:
[0061] Step S11: Obtain the target training data set.
[0062] In a specific implementation process, a target training data set needs to be obtained first, wherein the target training data set includes but not limited to a target image training set. When the target training data set is ...
PUM
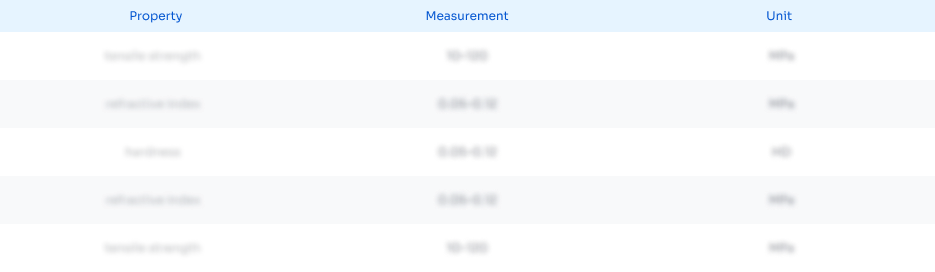
Abstract
Description
Claims
Application Information
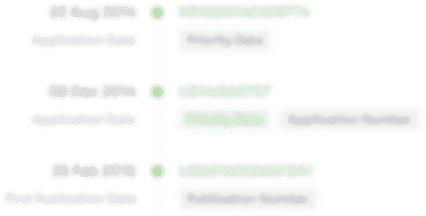
- R&D Engineer
- R&D Manager
- IP Professional
- Industry Leading Data Capabilities
- Powerful AI technology
- Patent DNA Extraction
Browse by: Latest US Patents, China's latest patents, Technical Efficacy Thesaurus, Application Domain, Technology Topic, Popular Technical Reports.
© 2024 PatSnap. All rights reserved.Legal|Privacy policy|Modern Slavery Act Transparency Statement|Sitemap|About US| Contact US: help@patsnap.com