Method, device, device and computer storage medium for training semantic representation model
A technology of semantic representation and training corpus, applied in the field of artificial intelligence, can solve the problems of high cost and difficulty in collecting enough corpus for training, and achieve the effect of reducing cost and high training efficiency
- Summary
- Abstract
- Description
- Claims
- Application Information
AI Technical Summary
Problems solved by technology
Method used
Image
Examples
Embodiment 1
[0030] figure 1 The flow chart of the method for training a semantic representation model provided in Embodiment 1 of the present application. The execution subject of this method is a device for training a semantic representation model. The device may be an application located in a computer system / server, or an application located in a computer system / server A functional unit such as a plug-in or a software development kit (Software Development Kit, SDK) in an application of the server. Such as figure 1 As shown in , the method may include the following steps:
[0031] In 101, acquire a semantic representation model that has been trained for a first language as a first semantic representation model.
[0032] Taking English as the first language as an example, since English is an international language, there are usually a lot of English corpus, so using English can easily and well train a semantic representation model, such as the Transformer model. In this step, the train...
Embodiment 2
[0049] In this embodiment, on the basis of the first embodiment, a semantic representation model trained in the first language is further obtained as a second semantic representation model. The first semantic representation model is used as the basis for layer-by-layer migration training, and the second semantic representation model is used to compare the results of the first language output by the second semantic representation model in the process of training the semantic representation model of the second language. The results output by the first semantic representation model are aligned.
[0050] Here, an additional alignment model needs to be added to assist the migration training of the first semantic representation model, and the alignment model is used to perform the above-mentioned alignment processing.
[0051] by figure 2 Take the training in stage (a) as an example, such as image 3 As shown in , the Chinese-English parallel corpus and the English training corpu...
Embodiment 3
[0069] Figure 5 The device structure diagram of the training semantic representation model provided for the third embodiment of the present application, such as Figure 5 As shown in , the device includes: a first acquisition unit 01 and a training unit 02, and may further include a second acquisition unit 03. The main functions of each component unit are as follows:
[0070] The first acquiring unit 01 is configured to acquire a semantic representation model that has been trained for the first language as a first semantic representation model.
[0071] The training unit 02 is used to use the bottom layer and top layer of the first semantic representation model as the trained layer, initialize the trained layer, keep the model parameters of other layers unchanged, and use the training corpus of the second language to train the trained layer , until the training end condition is reached; add the untrained layers to the trained layer one by one from bottom to top, and execute...
PUM
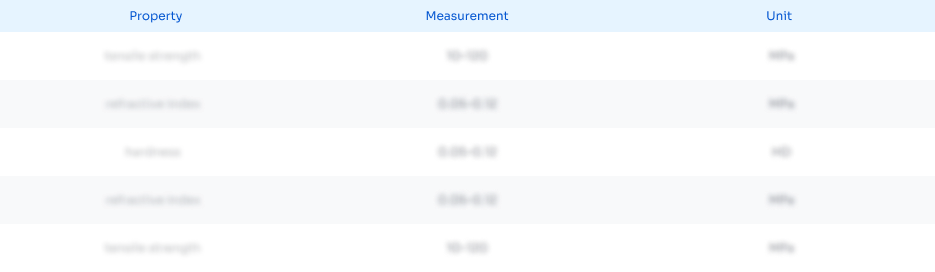
Abstract
Description
Claims
Application Information
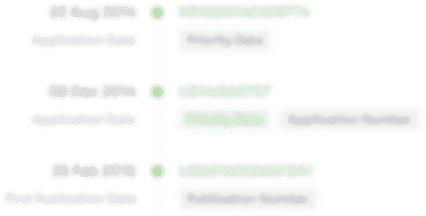
- R&D Engineer
- R&D Manager
- IP Professional
- Industry Leading Data Capabilities
- Powerful AI technology
- Patent DNA Extraction
Browse by: Latest US Patents, China's latest patents, Technical Efficacy Thesaurus, Application Domain, Technology Topic, Popular Technical Reports.
© 2024 PatSnap. All rights reserved.Legal|Privacy policy|Modern Slavery Act Transparency Statement|Sitemap|About US| Contact US: help@patsnap.com