High-resolution image landslide automatic detection method based on multi-level perception feature progressive self-learning
A high-resolution, multi-level technology, applied in neural learning methods, instruments, biological neural network models, etc., can solve problems such as irregular features, stagnant learning, and inability of machines to accurately learn image features. The effect of enhancing the ability to connect
- Summary
- Abstract
- Description
- Claims
- Application Information
AI Technical Summary
Problems solved by technology
Method used
Image
Examples
Embodiment Construction
[0034] Below in conjunction with accompanying drawing, technical scheme of the present invention is described in further detail:
[0035] Such as figure 1 As described above, the multi-level perceptual feature progressive self-learning method for landslide hazards based on high-resolution remote sensing images of the present invention includes the following steps 1-4.
[0036] Step 1. Divide feature levels based on perceptual depth. Using high-resolution images of the landslide area, based on perception depth-dependent image data and knowledge association, three feature levels are divided: visual perception layer, instrument perception layer and algorithm perception layer, and a three-layer perception feature set is obtained to support the progressive perception level. Feature normalization mapping; where the human eye's color perception of the image is the layered representation of the visual perception layer, the data characteristic perception recorded by the remote sensing...
PUM
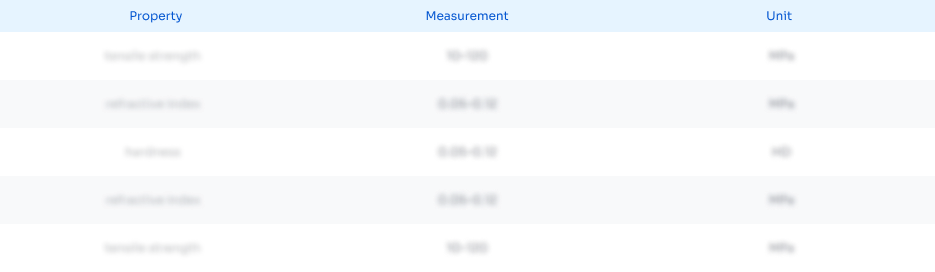
Abstract
Description
Claims
Application Information
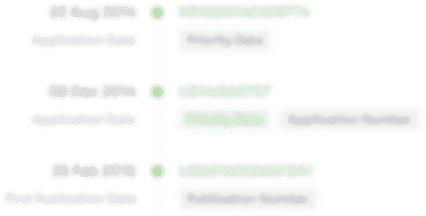
- R&D Engineer
- R&D Manager
- IP Professional
- Industry Leading Data Capabilities
- Powerful AI technology
- Patent DNA Extraction
Browse by: Latest US Patents, China's latest patents, Technical Efficacy Thesaurus, Application Domain, Technology Topic, Popular Technical Reports.
© 2024 PatSnap. All rights reserved.Legal|Privacy policy|Modern Slavery Act Transparency Statement|Sitemap|About US| Contact US: help@patsnap.com