Unsupervised clustering anomaly detection method
An unsupervised clustering and anomaly detection technology, applied in digital data information retrieval, special data processing applications, instruments, etc., can solve problems such as difficulty in acquiring knowledge and difficulty in building models
- Summary
- Abstract
- Description
- Claims
- Application Information
AI Technical Summary
Problems solved by technology
Method used
Image
Examples
Embodiment 1
[0089] Time scale alignment and equally spaced sample sequence generation;
[0090] Establish the Kth target telemetry data sequence with equal time intervals for the Kth telemetry data of the n original telemetry data, and obtain the Kth target telemetry data sequence through time scale alignment for the Kth target telemetry data sequence;
[0091] Assuming that there are n spacecraft telemetry parameters in total, record the original time series data of the kth telemetry parameter as {(t,s k (t)), t ∈ [t s ,t e ]}, where t represents time, s k (t) represents the data value of the kth telemetry parameter corresponding to time t, t s Indicates the start time, t e Indicates the end time.
[0092] In one embodiment, such as image 3 As shown, time scale alignment and equal interval sample sequence generation include the following step 101: given n spacecraft telemetry parameters, and sample sequence start time ts and end time t e , for a given time interval t d , satisfy...
Embodiment 2
[0103] 1. Modeling sample preparation;
[0104] In one embodiment, such as Figure 4 As shown, the Kth target telemetry data is standardized to obtain the data vector X of the target telemetry data. After time-scale alignment of each component of the data vector X of the Kth target telemetry data, the Single-Linkage clustering method Modeling to obtain a clustering model set S; modeling sample preparation includes the following steps:
[0105] 201: m sample data X with n telemetry parameters i ∈R n ,i=1,2,...,m,
[0106] where sample X i =(x i1 ,x i2 ,...,x in ),
[0107] Calculate the mean of each telemetry parameter sample data separately and standard deviation
[0108] 202: Use the Z-score method according to the formula Normalize the parameters, where x' ij is the standardized variable value, x ij is the actual variable value.
[0109] 203: Output the standardized data set D={X' i ,i=1,…,m}, where X i '=(x' i1 ,x′ i2 ,…,x′ in ).
[0110] 204: Use ra...
Embodiment 3
[0123] Calculate the detection threshold threshold;
[0124] In one embodiment such as Figure 6 Shown; Obtain the detection threshold threshold value step of the Kth target telemetry data as follows;
[0125] 401: Take a subset D of the data set D 2 .
[0126] 402: Calculate D 2 Each data vector in The distance from the clustering model set S Get the distance set {d i ,i=1,2,3,…,n}, n is the data set D 2 The number of data vectors in .
[0127] 403: Calculate distance set {d i ,i=1,2,3,…,n} average value and standard deviation
[0128] 404: Take the threshold value β=μ+3σ.
[0129] In dataset D 2 , calculate the detection threshold β=0.55 for data anomaly detection according to step 4.
PUM
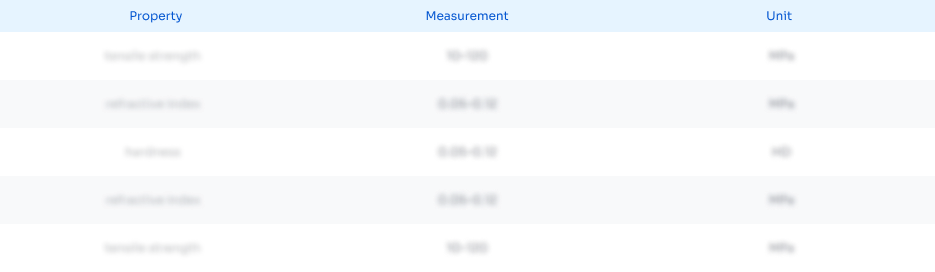
Abstract
Description
Claims
Application Information
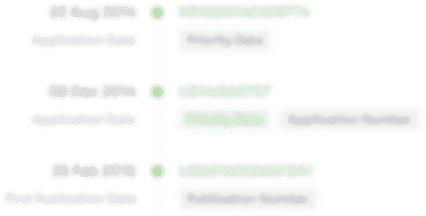
- R&D Engineer
- R&D Manager
- IP Professional
- Industry Leading Data Capabilities
- Powerful AI technology
- Patent DNA Extraction
Browse by: Latest US Patents, China's latest patents, Technical Efficacy Thesaurus, Application Domain, Technology Topic, Popular Technical Reports.
© 2024 PatSnap. All rights reserved.Legal|Privacy policy|Modern Slavery Act Transparency Statement|Sitemap|About US| Contact US: help@patsnap.com