Semi-supervised hyperspectral data analysis method based on double-flow conditional generative adversarial network
A data analysis and hyperspectral technology, applied in the field of hyperspectral detection, which can solve the problems of limited number of training samples, poor model accuracy and generalization ability.
- Summary
- Abstract
- Description
- Claims
- Application Information
AI Technical Summary
Problems solved by technology
Method used
Image
Examples
Embodiment Construction
[0068] In order to make the above objects, features and advantages of the present invention more comprehensible, the technical solutions of the present invention will be described in detail below in conjunction with the accompanying drawings and specific embodiments. It should be pointed out that the described embodiments are only a part of the embodiments of the present invention, rather than all embodiments. Based on the embodiments of the present invention, all those skilled in the art can obtain without creative work. Other embodiments all belong to the protection scope of the present invention.
[0069] This embodiment uses hyperspectral measurement to measure the content of soluble solid solution in strawberries. Such as figure 1 As shown, a semi-supervised hyperspectral data quantitative analysis method based on two-stream conditional confrontation generation network, the specific steps are as follows:
[0070] S1. Obtaining hyperspectral sample data: A total of 406 s...
PUM
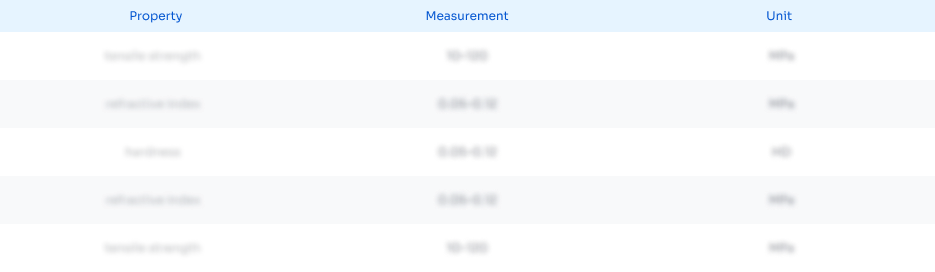
Abstract
Description
Claims
Application Information
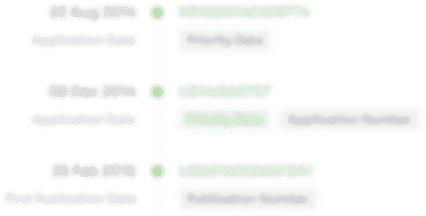
- R&D Engineer
- R&D Manager
- IP Professional
- Industry Leading Data Capabilities
- Powerful AI technology
- Patent DNA Extraction
Browse by: Latest US Patents, China's latest patents, Technical Efficacy Thesaurus, Application Domain, Technology Topic, Popular Technical Reports.
© 2024 PatSnap. All rights reserved.Legal|Privacy policy|Modern Slavery Act Transparency Statement|Sitemap|About US| Contact US: help@patsnap.com