A fast semi-supervised classification method based on graph volume positive learning machine
A classification method and learning machine technology, applied in the field of fast semi-supervised classification, can solve problems such as slow convergence speed and falling into local optimum, and achieve the effect of maintaining a fast learning speed
- Summary
- Abstract
- Description
- Claims
- Application Information
AI Technical Summary
Problems solved by technology
Method used
Image
Examples
Embodiment Construction
[0046] In order to make the purpose, technical solution and advantages of the present invention clearer, the embodiments of the present invention will be further described below in conjunction with the accompanying drawings.
[0047] Please refer to figure 1 , the embodiment of the present invention provides a framework diagram of a fast semi-supervised classification method based on a graph volume positive learning machine, specifically including:
[0048] S101: Construct a self-expression model of the disease classification data, and use the self-expression model to construct a global robust map of the disease classification data, and obtain an adjacency matrix A of the disease classification data;
[0049] S102: Calculate the random graph convolution model output H according to the adjacency matrix A;
[0050] S103: Combined with the extreme learning machine, according to the output H of the random graph convolution model, calculate the output layer weight β of the graph c...
PUM
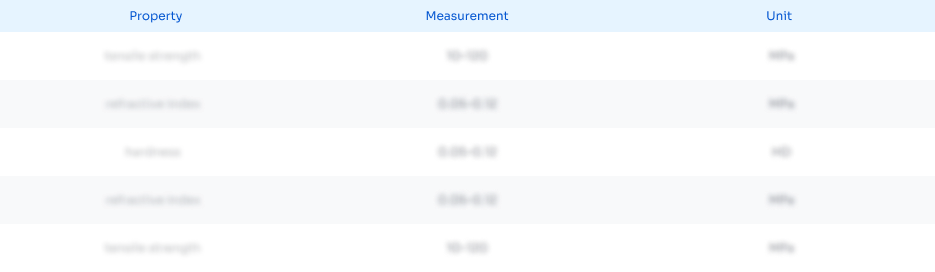
Abstract
Description
Claims
Application Information
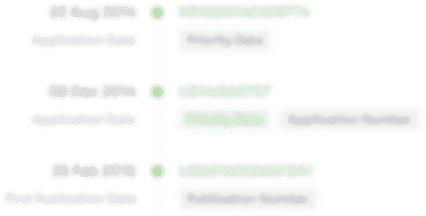
- R&D
- Intellectual Property
- Life Sciences
- Materials
- Tech Scout
- Unparalleled Data Quality
- Higher Quality Content
- 60% Fewer Hallucinations
Browse by: Latest US Patents, China's latest patents, Technical Efficacy Thesaurus, Application Domain, Technology Topic, Popular Technical Reports.
© 2025 PatSnap. All rights reserved.Legal|Privacy policy|Modern Slavery Act Transparency Statement|Sitemap|About US| Contact US: help@patsnap.com