Equipment fault defect diagnosis method based on improved U-Net neural network
A technology for equipment failure and defect diagnosis, which is applied in the field of equipment appearance failure and defect crack diagnosis. It can solve problems such as slow training speed, inability to achieve pixel-level positioning accuracy in complex backgrounds, and increased memory overhead.
- Summary
- Abstract
- Description
- Claims
- Application Information
AI Technical Summary
Problems solved by technology
Method used
Image
Examples
Embodiment Construction
[0081] The present invention will be further described below in conjunction with accompanying drawing.
[0082] The invention is based on the improved U-Net neural network equipment failure defect diagnosis method, mainly including three major functional modules: image enhancement, 3D display based on the improved U-Net neural network model and fault location and shape. Among them, in order to solve the problem that the original appearance image of the device may not be directly used for network training due to the uncertainty of the source, the size, quantity, and image quality, the original image is adjusted by size adjustment, flipping, and angle rotation. The size, quantity, shape, etc. of the image are processed by the front-end, and the original image set is optimized by means of image enhancement, and then the optimized sample is put into the improved U-Net neural network model for training, and finally the output is the same size as the original image. Pixel-level imag...
PUM
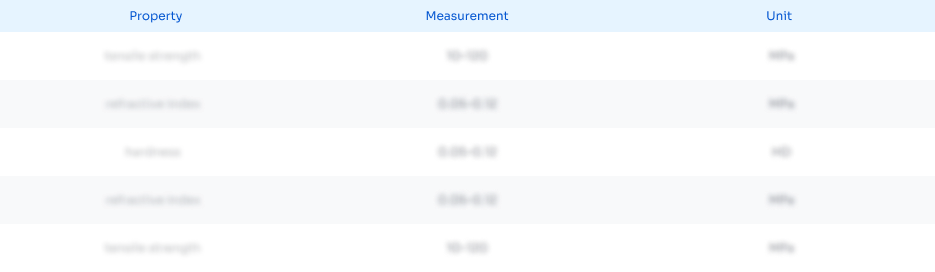
Abstract
Description
Claims
Application Information
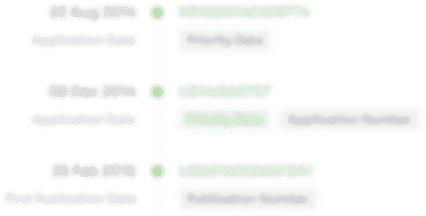
- R&D Engineer
- R&D Manager
- IP Professional
- Industry Leading Data Capabilities
- Powerful AI technology
- Patent DNA Extraction
Browse by: Latest US Patents, China's latest patents, Technical Efficacy Thesaurus, Application Domain, Technology Topic, Popular Technical Reports.
© 2024 PatSnap. All rights reserved.Legal|Privacy policy|Modern Slavery Act Transparency Statement|Sitemap|About US| Contact US: help@patsnap.com