Remote sensing image scene classification method based on multi-similarity measurement deep learning
A similarity measurement and remote sensing image technology, applied in the field of remote sensing image scene classification, can solve the problems of inability to fully express the differences between different classes, single similarity, redundant information, etc.
- Summary
- Abstract
- Description
- Claims
- Application Information
AI Technical Summary
Problems solved by technology
Method used
Image
Examples
Embodiment Construction
[0081] The technical solutions of the present invention will be further described below in conjunction with the accompanying drawings and embodiments.
[0082] Such as figure 1 Shown, technical scheme of the present invention is described in further detail as follows:
[0083] (1) A deep convolutional neural network is used to train remote sensing scene images, and a multiple similarity loss is added to the traditional cross-entropy loss to form a new loss function. The multiple similarity loss item maps the extracted fully connected features to the metric distance space, calculates and constrains the metric distance between input image samples, so that the network model is more discriminative, and can effectively classify remote sensing scene images.
[0084] (1.1) Build X = {xi |i=1,2,...,N} is the input remote sensing image data sample, Y={y i |i=1,2,...,N} is the category label set corresponding to the input remote sensing image data sample, y i ∈ R c Indicates the lab...
PUM
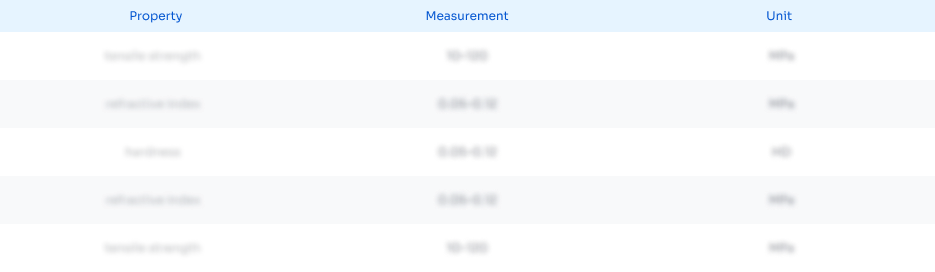
Abstract
Description
Claims
Application Information
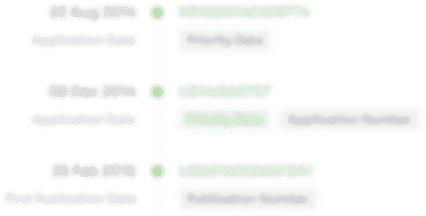
- R&D Engineer
- R&D Manager
- IP Professional
- Industry Leading Data Capabilities
- Powerful AI technology
- Patent DNA Extraction
Browse by: Latest US Patents, China's latest patents, Technical Efficacy Thesaurus, Application Domain, Technology Topic, Popular Technical Reports.
© 2024 PatSnap. All rights reserved.Legal|Privacy policy|Modern Slavery Act Transparency Statement|Sitemap|About US| Contact US: help@patsnap.com