Image restoration model training method and system and image restoration method
A technology for repairing models and training methods, which is applied in the field of image repair, and can solve problems such as unnatural areas, difficult repairs, and poor repair effects
- Summary
- Abstract
- Description
- Claims
- Application Information
AI Technical Summary
Problems solved by technology
Method used
Image
Examples
Embodiment 1
[0060] Example 1: Model training
[0061] Step 1 data preprocessing.
[0062] Step 1.1 Specify the number of masks (NUM_MASK), the maximum number of inflection points of the mask (NUM_VER), the minimum distance between two pixels (MIN_LEN), the maximum distance between two pixels (MAX_LEN), two pixels The minimum width of a line segment between points (MIN_BRUSH), the maximum width of a line segment between two pixel points (MAX_BRUSH), and the maximum corner (MAX_ANG) are 3, 5, 50, 200, 10, 40, and 20, respectively.
[0063] Step 1.1 Random mask hyperparameter setting.
[0064] For each image in the dataset, generate an image with the same resolution and a black background. For each image, randomly generate a number from 1 to 3 to represent the number of generated masks. For each mask generation process, a number from 1 to 5 is randomly generated to represent the number of inflection points of the mask.
[0065] Step 1.2 Randomly initialize a pixel within the image resolu...
Embodiment 2
[0086] Example 2: Model Reasoning
[0087] In the model reasoning stage, use the flow as attached Figure 5 shown.
[0088] Step 1 Image collection. In the image restoration stage, users need to select images with objects or targets that are unclear or incomplete according to their own needs.
[0089]Step 2 Prior information. In the prior information stage, the user needs to mark the blurred or incomplete areas in the image on the original image, and the marked area needs to cover the blurred or incomplete areas as much as possible. Afterwards, the user needs to perform a second type of labeling on the edge of the original object in the labeling area. If the edge of the object is blurred or incomplete, users can mark the edge according to their own understanding.
[0090] Step 3 generates mask image and edge information image. In the stage of generating the mask image, according to the user's first type of annotation, the user's annotation area will be drawn on a white b...
PUM
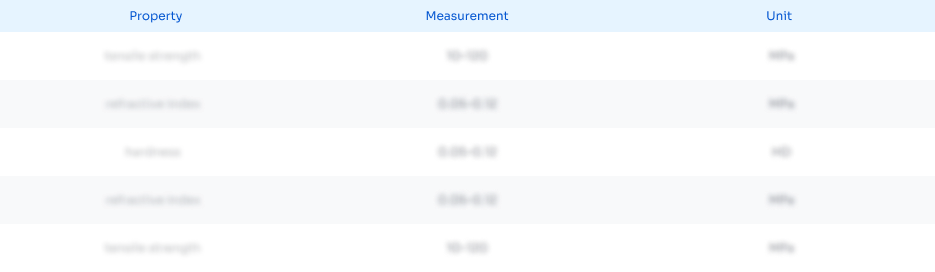
Abstract
Description
Claims
Application Information
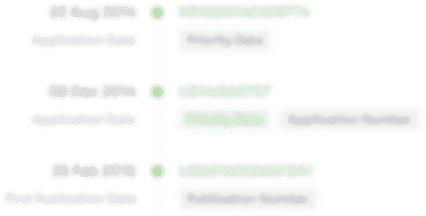
- R&D Engineer
- R&D Manager
- IP Professional
- Industry Leading Data Capabilities
- Powerful AI technology
- Patent DNA Extraction
Browse by: Latest US Patents, China's latest patents, Technical Efficacy Thesaurus, Application Domain, Technology Topic, Popular Technical Reports.
© 2024 PatSnap. All rights reserved.Legal|Privacy policy|Modern Slavery Act Transparency Statement|Sitemap|About US| Contact US: help@patsnap.com