Network representation learning method based on meta-structure and graph neural network
A neural network and learning method technology, applied in neural learning methods, biological neural network models, other database retrieval based on metadata, etc., can solve problems such as excessive singleness and increase the difficulty of mechanism operation, and achieve excellent results.
- Summary
- Abstract
- Description
- Claims
- Application Information
AI Technical Summary
Problems solved by technology
Method used
Image
Examples
Embodiment Construction
[0015] In order to make the technical means, creative features, goals and effects of the present invention easy to understand, a network representation learning method based on the meta-structure and graph neural network of the present invention will be described in detail below in conjunction with the embodiments and accompanying drawings.
[0016] The network representation learning method provided by the present invention is based on meta-structure and graph neural network, specifically, a hierarchical search algorithm is proposed to generate a meta-structure, and the meta-structure can make up for the deficiency of meta-paths in capturing heterogeneous information network relationships; for different meta The structure is learned through independent parameters of different graph neural networks, and the final graph neural network can automatically learn the important meta-structure for each node; after that, the node representations obtained for different meta-structures are...
PUM
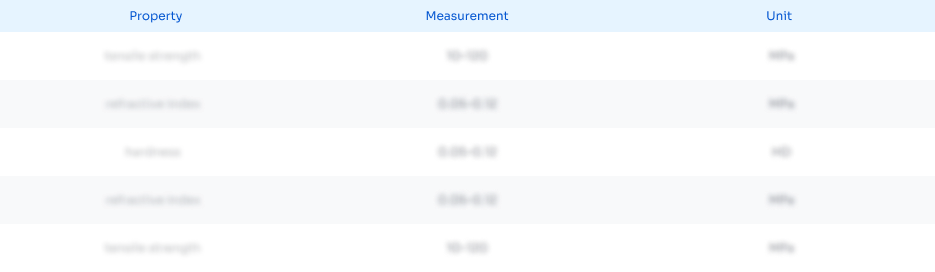
Abstract
Description
Claims
Application Information
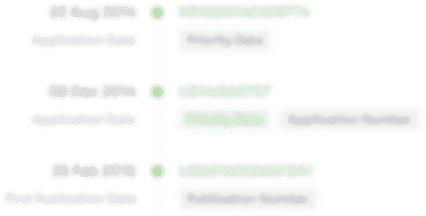
- R&D
- Intellectual Property
- Life Sciences
- Materials
- Tech Scout
- Unparalleled Data Quality
- Higher Quality Content
- 60% Fewer Hallucinations
Browse by: Latest US Patents, China's latest patents, Technical Efficacy Thesaurus, Application Domain, Technology Topic, Popular Technical Reports.
© 2025 PatSnap. All rights reserved.Legal|Privacy policy|Modern Slavery Act Transparency Statement|Sitemap|About US| Contact US: help@patsnap.com