Hyperspectral image classification method based on double-branch convolution auto-encoder
A technology of convolutional auto-encoder and hyperspectral image, which is applied in the field of hyperspectral image classification based on double-branch convolutional autoencoder, and can solve the problem of low classification accuracy.
- Summary
- Abstract
- Description
- Claims
- Application Information
AI Technical Summary
Problems solved by technology
Method used
Image
Examples
Embodiment Construction
[0029] The present invention will be described in further detail below in conjunction with the accompanying drawings and specific embodiments.
[0030] refer to figure 1 , the present invention comprises the following steps:
[0031] Step 1) Obtain a supervised training sample set S of hyperspectral data n , Supervised training sample label vector set Unsupervised training sample set S u and test sample set S t :
[0032] (1a) Input a hyperspectral image with a size of W×H×B and the corresponding label map with a size of W×H, W and H represent the number of rows and columns of pixels in the hyperspectral image and label map, and B represents the height The number of bands of the spectral image, in this example, the input hyperspectral image is the Indian Pines hyperspectral image, W=145, H=145, B=220;
[0033] (1b) Taking the same point in the hyperspectral image as the position of each pixel whose value is not 0 in the label map as the center, delineate the size as W ...
PUM
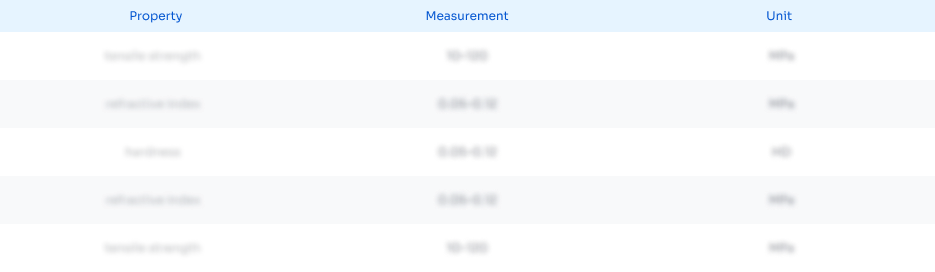
Abstract
Description
Claims
Application Information
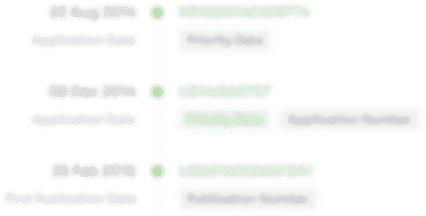
- R&D Engineer
- R&D Manager
- IP Professional
- Industry Leading Data Capabilities
- Powerful AI technology
- Patent DNA Extraction
Browse by: Latest US Patents, China's latest patents, Technical Efficacy Thesaurus, Application Domain, Technology Topic, Popular Technical Reports.
© 2024 PatSnap. All rights reserved.Legal|Privacy policy|Modern Slavery Act Transparency Statement|Sitemap|About US| Contact US: help@patsnap.com