Remote sensing hyperspectral image classification method based on local binary pattern and KNN classifier
A local binary mode, hyperspectral image technology, applied in the field of remote sensing hyperspectral images, can solve difficult remote sensing hyperspectral image classification and other problems
- Summary
- Abstract
- Description
- Claims
- Application Information
AI Technical Summary
Problems solved by technology
Method used
Image
Examples
Embodiment 1
[0174] In order to verify the impact of different parameter settings on the classification accuracy in the method proposed by the present invention, the number p of the principal components, the neighborhood radius r of the LBP algorithm, the number of neighboring sampling points s, the size w of the divided local area, the classification The neighbor number k of the device is verified on these five parameters. In order to improve the accuracy and reliability of the experiment, each experiment was repeated 10 times, each time 10% of the training samples were randomly selected from each type of ground object samples, and the rest were used as test samples, and the classification accuracy of the 10 experiments was averaged. to the final result.
[0175] Table 4 Comparison table of variance contribution rate and cumulative contribution rate of each principal component in different data sets
[0176]
[0177] Figure 5 It is the OA dot-line diagram of the classification metho...
Embodiment 2
[0181] In order to further verify the effectiveness of the method proposed in the present invention, three data sets of Pavia University, Indian Pines, and Salinas will be used for verification, and the classification method LBP-MFKNN described in the present invention will be compared with some existing hyperspectral classifications The methods KNN, RBF-SVM and KSOMP are compared, and the number of training samples used by each classification method is exactly the same.
[0182] Table 6 Comparison results of the classification accuracy of the classification method LBP-MFKNN described in the present invention and the existing method on the Pavia University dataset.
[0183]
[0184] right figure 2 The shown Pavia University hyperspectral image is classified, and 10% samples are randomly selected as a training set from each type of ground object, and the remaining samples are used as a test set. In the classification method proposed by the present invention on this data set...
Embodiment 3
[0192] In order to verify the impact of the classification method of the present invention on the hyperspectral classification accuracy under different sample ratios, five different training sample ratios of 1%, 2%, 5%, 10%, 15%, and 20% were set up for verification , the classification method LBP-MFKNN described in the present invention is to Pavia University, Indian Pines, Salinas data set OA, AA, Kappa experiment result under different sample proportions respectively as follows Figure 11 (a,b,c), Figure 11 (d, e, f), Figure 11 (g, h, i). From Figure 11 It can be seen that with the continuous increase of the training sample size, the classification accuracy of different classification methods is also continuously improved, and gradually tends to be stable. Under various training sample sizes, the classification method that combines more features has higher OA, AA, and Kappa coefficients than the classification method that only uses a single feature in most cases. Whe...
PUM
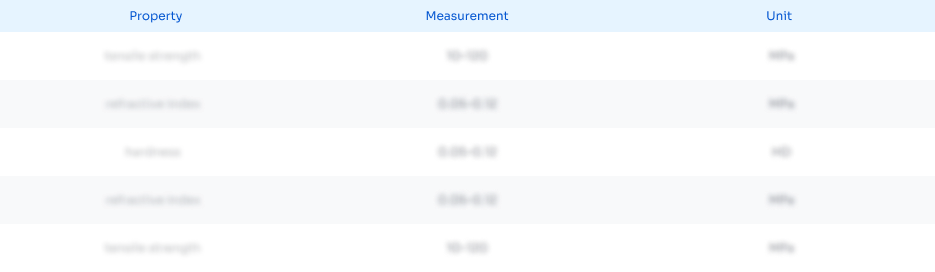
Abstract
Description
Claims
Application Information
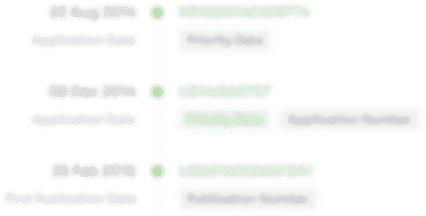
- R&D Engineer
- R&D Manager
- IP Professional
- Industry Leading Data Capabilities
- Powerful AI technology
- Patent DNA Extraction
Browse by: Latest US Patents, China's latest patents, Technical Efficacy Thesaurus, Application Domain, Technology Topic, Popular Technical Reports.
© 2024 PatSnap. All rights reserved.Legal|Privacy policy|Modern Slavery Act Transparency Statement|Sitemap|About US| Contact US: help@patsnap.com