Lung parenchyma CT image segmentation method based on weighted full convolutional neural network
A convolutional neural network and CT image technology, applied in the field of medical image processing, can solve problems such as incorrect segmentation, improve reliability, improve semantic segmentation performance, and reduce model redundant calculations
- Summary
- Abstract
- Description
- Claims
- Application Information
AI Technical Summary
Problems solved by technology
Method used
Image
Examples
Embodiment Construction
[0049] The present invention can automatically process lung CT images and extract lung parenchymal regions; the present invention uses new technical means to improve the segmentation accuracy of lesion regions in lung parenchyma, and provide more reliable information support for clinical diagnosis.
[0050] Such as process figure 1 As shown, a deep convolutional neural network lung parenchymal segmentation method based on a weighted loss function includes the following 6 steps:
[0051] 1. Select LUNA16 (Lung Nodule Analysis 16, https: / / luna16.grand-challenge.org / ) lung CT image data set for preprocessing, and make model training and test data sets;
[0052] 2. Design a deep convolutional neural network based on the standard FCN network framework, and use the standard encoding-decoding path structure to include the principles of jump connection, dilation convolution and batch normalization, and establish the overall structure of the lung parenchymal segmentation convolutional neural n...
PUM
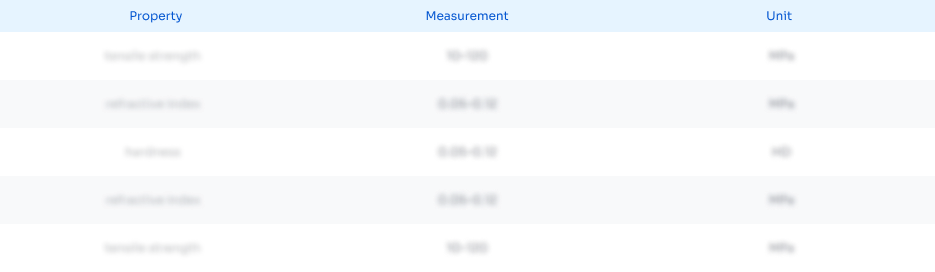
Abstract
Description
Claims
Application Information
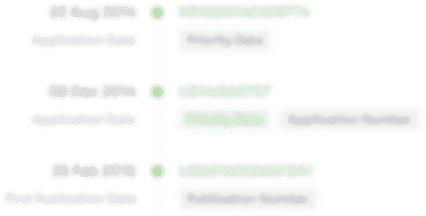
- R&D Engineer
- R&D Manager
- IP Professional
- Industry Leading Data Capabilities
- Powerful AI technology
- Patent DNA Extraction
Browse by: Latest US Patents, China's latest patents, Technical Efficacy Thesaurus, Application Domain, Technology Topic.
© 2024 PatSnap. All rights reserved.Legal|Privacy policy|Modern Slavery Act Transparency Statement|Sitemap