Detection method for eyeground multi-disease classification based on deep learning
A deep learning and detection method technology, applied in the field of medical image processing, can solve problems such as low detection efficiency and complex models, and achieve the effects of high precision, fast speed, improved efficiency and accuracy
- Summary
- Abstract
- Description
- Claims
- Application Information
AI Technical Summary
Problems solved by technology
Method used
Image
Examples
Embodiment 1
[0027] Implementation Example 1: The detection method for the classification of multiple fundus diseases based on deep learning provided by the present invention firstly performs preprocessing and data enhancement on the input fundus image to improve the robustness of the network, and then performs feature extraction and Attention Module through EfficientNet Carry out feature enhancement, use the fully connected layer for classification judgment, and finally output the classification probabilities, such as figure 1 As shown, the detection method of fundus multi-disease classification based on deep learning includes the following steps:
[0028] Step 1: Select a dataset. The data sets used in the present invention are selected from a total of 36,910 fundus color photo data provided by Nanjing Mingji Hospital, Jiangsu Provincial Government Hospital and Jiangsu Provincial People's Hospital. The data of each disease are: normal: 17152; high myopia: 7475; other abnormalities: 3990...
PUM
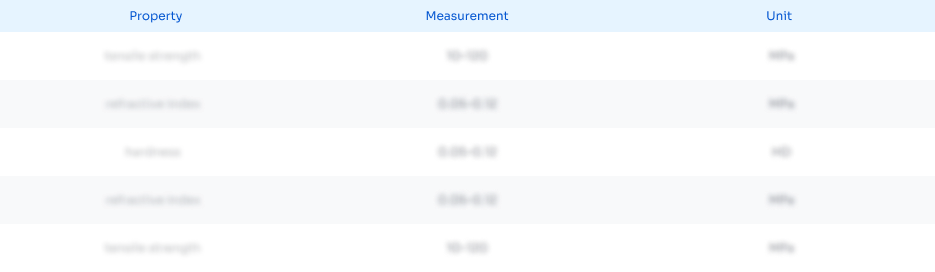
Abstract
Description
Claims
Application Information
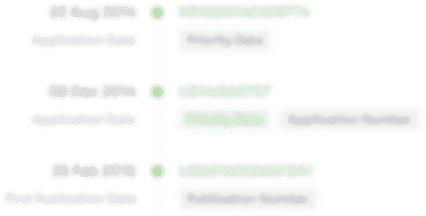
- R&D Engineer
- R&D Manager
- IP Professional
- Industry Leading Data Capabilities
- Powerful AI technology
- Patent DNA Extraction
Browse by: Latest US Patents, China's latest patents, Technical Efficacy Thesaurus, Application Domain, Technology Topic.
© 2024 PatSnap. All rights reserved.Legal|Privacy policy|Modern Slavery Act Transparency Statement|Sitemap