Real-time multi-scale target detection method based on lightweight convolutional neural network
A convolutional neural network and target detection technology, applied in the field of real-time multi-scale target detection, can solve problems such as low detection accuracy, and achieve the effects of improving detection accuracy, reducing model complexity, and reducing computational complexity
- Summary
- Abstract
- Description
- Claims
- Application Information
AI Technical Summary
Problems solved by technology
Method used
Image
Examples
Embodiment
[0035] figure 1 A network structure diagram of a real-time multi-scale target detection method based on a lightweight convolutional neural network disclosed in the present invention is given. The method specifically includes the following steps:
[0036] Step T1. Use the K-Means clustering algorithm to cluster the height-to-width ratios of all objects in the training data set samples, and use the cluster center as the height-to-width ratio of the anchor box; after determining the height-to-width ratio, use the K-Means clustering algorithm The area scale coefficient of the feature map of each layer of hierarchical clustering, the cluster center is used as the scale coefficient of the anchor box of the corresponding layer;
[0037] First, the aspect ratio of the target frame of the training data set sample is counted, and the target frame with the smallest Th% aspect ratio and the Th% target frame with the largest aspect ratio are removed to prevent the abnormal aspect ratio fro...
PUM
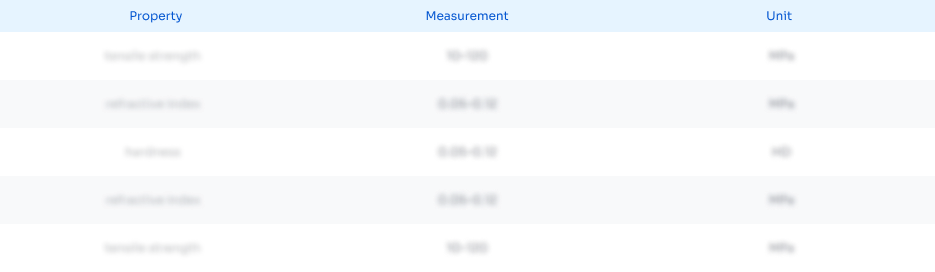
Abstract
Description
Claims
Application Information
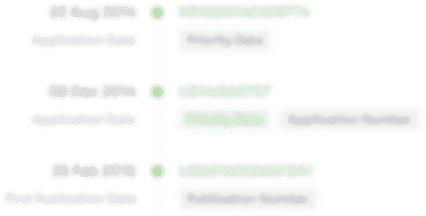
- R&D
- Intellectual Property
- Life Sciences
- Materials
- Tech Scout
- Unparalleled Data Quality
- Higher Quality Content
- 60% Fewer Hallucinations
Browse by: Latest US Patents, China's latest patents, Technical Efficacy Thesaurus, Application Domain, Technology Topic, Popular Technical Reports.
© 2025 PatSnap. All rights reserved.Legal|Privacy policy|Modern Slavery Act Transparency Statement|Sitemap|About US| Contact US: help@patsnap.com