Stack type self-encoding multi-model load prediction method and system based on LSTM
A technology of stacked self-encoding and prediction method, which is applied in the field of multi-model load prediction based on LSTM stacked self-encoding, which can solve the problems of destroying the hidden value inside the data and failing to make full use of multi-variable time series data, etc.
- Summary
- Abstract
- Description
- Claims
- Application Information
AI Technical Summary
Problems solved by technology
Method used
Image
Examples
Embodiment Construction
[0188] The present invention will be further described below with reference to the accompanying drawings:
[0189] The present invention proposes a method and system based on LSTM stack type self-coding multi-model load prediction method and system. First, the data set data is pretreated to achieve noise reduction and normalization. Secondly construct the probability model of the electric vehicle charging start time, standardization treatment makes it a temporal influence factor, inserts a data set. The LSTM stamp encoder structure is then constructed to implement the feature extraction of the input sequence. Finally, the reconstructed input sequence is entered into the XGBoost model to obtain the forecast results. Compared with the conventional short-term load prediction method, the present invention can sufficiently excavate the multivariate timing historical data, and the generalization capacity is strong and effectively improves short-term load pre-measurement.
[0190] The pr...
PUM
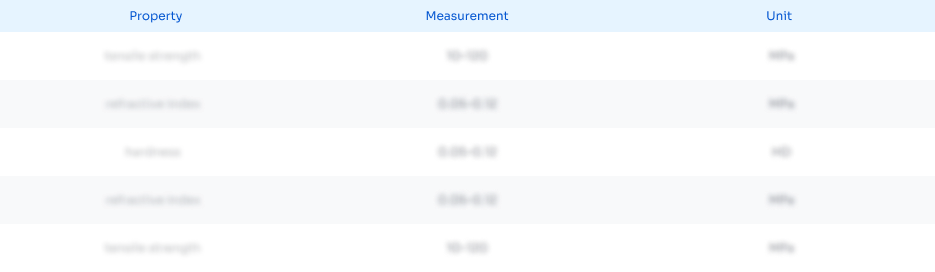
Abstract
Description
Claims
Application Information
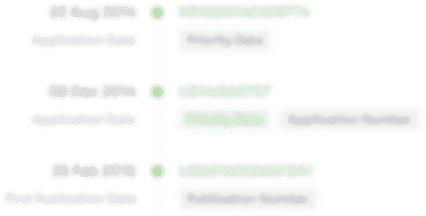
- Generate Ideas
- Intellectual Property
- Life Sciences
- Materials
- Tech Scout
- Unparalleled Data Quality
- Higher Quality Content
- 60% Fewer Hallucinations
Browse by: Latest US Patents, China's latest patents, Technical Efficacy Thesaurus, Application Domain, Technology Topic, Popular Technical Reports.
© 2025 PatSnap. All rights reserved.Legal|Privacy policy|Modern Slavery Act Transparency Statement|Sitemap|About US| Contact US: help@patsnap.com