Mechanical equipment multi-working-condition fault prediction method based on attention mechanism
A technology for mechanical equipment and fault prediction, applied in prediction, neural learning methods, instruments, etc., can solve problems such as time-consuming and labor-intensive, failure to find operating conditions, failure to make full use of sensor data time dependence, etc., to improve convergence Effects of Speed and Accuracy
- Summary
- Abstract
- Description
- Claims
- Application Information
AI Technical Summary
Problems solved by technology
Method used
Image
Examples
Embodiment Construction
[0049] Below in conjunction with accompanying drawing, technical scheme of the present invention is described in further detail:
[0050] Such as Figure 1-2 As shown, this embodiment proposes a multi-condition fault prediction method for mechanical equipment based on the attention mechanism, including the following steps:
[0051] Step 1: Take out the original data from the database received and saved on the sensor, and preprocess the original data; first, receive the original data and vectorize it, recorded as T c is the failure cycle of the cth component, c∈{1,2,…,n}; each item is a K+1-dimensional vector {x 1 ,x 2 ,...,x k ,y i}, respectively represent k sensor input eigenvalues and corresponding RUL values; then normalize the data, the calculation formula is That is, the corresponding normalized value of each item in S is Finally, the data is time-divided to form sliding time window data, that is, O={o i |i>=0, ic -L}, where o i =s i 'The window length is L...
PUM
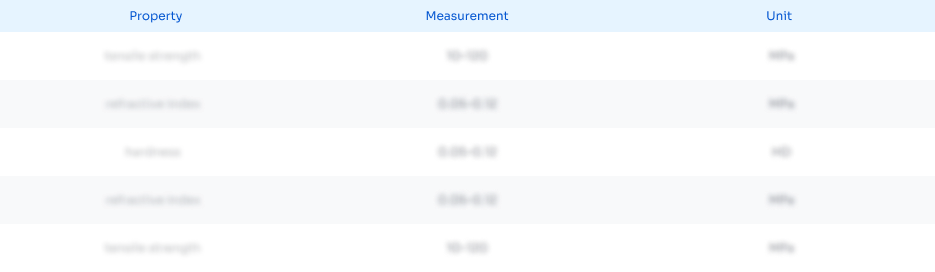
Abstract
Description
Claims
Application Information
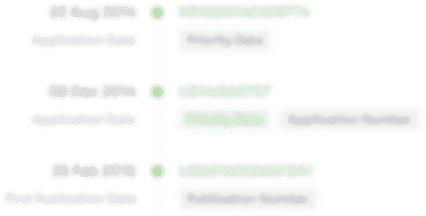
- R&D
- Intellectual Property
- Life Sciences
- Materials
- Tech Scout
- Unparalleled Data Quality
- Higher Quality Content
- 60% Fewer Hallucinations
Browse by: Latest US Patents, China's latest patents, Technical Efficacy Thesaurus, Application Domain, Technology Topic, Popular Technical Reports.
© 2025 PatSnap. All rights reserved.Legal|Privacy policy|Modern Slavery Act Transparency Statement|Sitemap|About US| Contact US: help@patsnap.com