Commodity recommendation method and system based on deep neural network
A deep neural network, product recommendation technology, applied in the field of intelligent recommendation, can solve the problems of inability to solve the recommendation algorithm, simple neural network gradient disappearance, gradient explosion, etc., to avoid the recommendation of non-related products, reduce the recommendation of non-related products, solve Effects of Gradient Problems
- Summary
- Abstract
- Description
- Claims
- Application Information
AI Technical Summary
Problems solved by technology
Method used
Image
Examples
Embodiment
[0092] A product recommendation algorithm based on deep neural network is divided into five steps as follows: figure 1 As shown, the first step is the input layer, the stage of user and product feature acquisition; the second step is the embedding layer, the initial processing stage of user and product features; the third step is the user-product interaction layer, user features and product features The interaction stage; the fourth step is the residual network layer, which is the stage of deep extraction of latent features. The deep residual network is used to solve the problem that MLP in the neural matrix model is difficult to capture highly complex nonlinear latent features in user-commodity interaction data; The five steps are the output layer, the user's desired product prediction stage, and the sigmoid function is used as the activation function to predict the user's desired product recommendation.
[0093] The specific steps are as follows:
[0094] Step 1: Input lay...
PUM
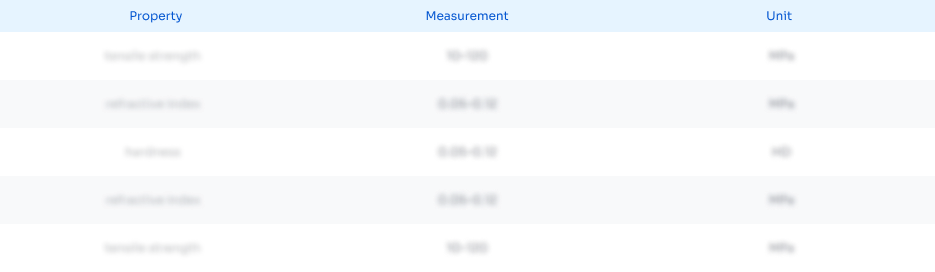
Abstract
Description
Claims
Application Information
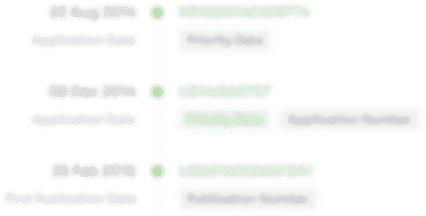
- R&D Engineer
- R&D Manager
- IP Professional
- Industry Leading Data Capabilities
- Powerful AI technology
- Patent DNA Extraction
Browse by: Latest US Patents, China's latest patents, Technical Efficacy Thesaurus, Application Domain, Technology Topic, Popular Technical Reports.
© 2024 PatSnap. All rights reserved.Legal|Privacy policy|Modern Slavery Act Transparency Statement|Sitemap|About US| Contact US: help@patsnap.com