Rolling bearing fault identification method based on GAF-CNN-BiGRU network
A technology for rolling bearing and fault identification, applied in the field of rolling bearing fault identification based on GAF-CNN-BiGRU network, rolling bearing fault identification, can solve the problems of limited feature information, increase the complexity of image conversion, time-dependent feature extraction, etc., to improve accuracy The effect of rate, retention dependence
- Summary
- Abstract
- Description
- Claims
- Application Information
AI Technical Summary
Problems solved by technology
Method used
Image
Examples
Embodiment 1
[0064] This embodiment uses inner ring fault data to explain in detail the rolling bearing fault identification method based on the GAF-CNN-BiGRU network provided by the present invention.
[0065] In this embodiment, the vibration signals and normal vibration signals of three kinds of damage diameters (mild 0.007inch, moderate 0.014inch and severe 0.021inch) at the same fault location of the drive end bearing at a sampling frequency of 12kHz are selected as the research objects. The length is 864 sampling points for data division. In order to obtain enough data for training, the data in the collected data set is first enhanced by overlapping samples to expand the number of training samples. Partial overlap, which not only ensures the full use of the signal, but also further expands the number of samples. In this way, a total of 4000 sample data are obtained to form the original data set.
[0066] In this embodiment, 2400 sample data are used for model training in the origin...
Embodiment 2
[0111] In order to further verify the feasibility of the rolling bearing fault identification method based on the GAF-CNN-BiGRU network provided by the present invention, this embodiment further selects 12kHz sampling frequency, the driving end bearing is the same as three different fault locations (inner ring, rolling element and outer ring) and the same fault degree (damage diameter of 0.014inch) and normal vibration signals are used as research objects. Obtain the training set, verification set and test set for model training according to the same data processing method as in Example 1. Then train the CNN-BiGRU network model according to the training method provided in Steps S1-S4 in Embodiment 1.
[0112] Then use the trained CNN-BiGRU network model to identify rolling bearing faults on the test set data according to the same identification method as steps L1-L2 in Example 1. And use the same data set to train and test through the common deep learning algorithm CNN, deep ...
PUM
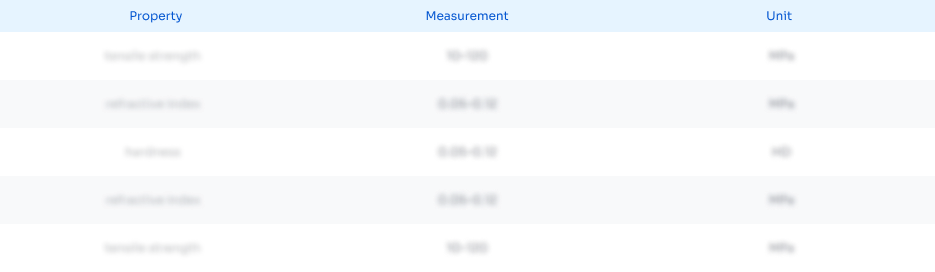
Abstract
Description
Claims
Application Information
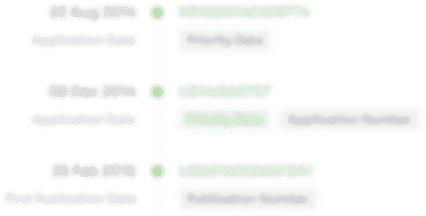
- R&D Engineer
- R&D Manager
- IP Professional
- Industry Leading Data Capabilities
- Powerful AI technology
- Patent DNA Extraction
Browse by: Latest US Patents, China's latest patents, Technical Efficacy Thesaurus, Application Domain, Technology Topic, Popular Technical Reports.
© 2024 PatSnap. All rights reserved.Legal|Privacy policy|Modern Slavery Act Transparency Statement|Sitemap|About US| Contact US: help@patsnap.com