Power grid section data retrieval method considering manifold sorting algorithm
A manifold sorting and data retrieval technology, applied in digital data information retrieval, electrical digital data processing, special data processing applications, etc., to avoid the problem of dimension disaster, improve accuracy, and improve similarity measurement.
- Summary
- Abstract
- Description
- Claims
- Application Information
AI Technical Summary
Problems solved by technology
Method used
Image
Examples
Embodiment 1
[0026] Embodiment 1, a grid section data query and retrieval method based on manifold sorting, the method is composed of five parts: use the low-dimensional manifold subspace for data retrieval, and describe the grid section data as corresponding Points, create a weighted graph model, improve the original similarity measure based on Euclidean distance, use belief propagation to assign ranking scores, and improve the accuracy of retrieval results. The present invention will be further described below using the accompanying drawings and examples.
[0027] (1) Describe the grid section data as corresponding points in the multidimensional vector space
[0028] Map the power data in the dataset to the corresponding points in the vector space and create a K-NN graph.
[0029] (2) Create a weighted graph model
[0030] Calculate node x in K-NN graph i and x j The weight W of the edge between ij , if no edge exists, then W ij =0, so as to obtain the weight matrix; normalize the we...
PUM
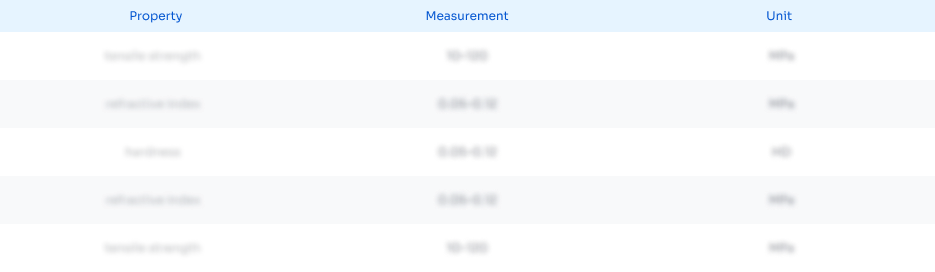
Abstract
Description
Claims
Application Information
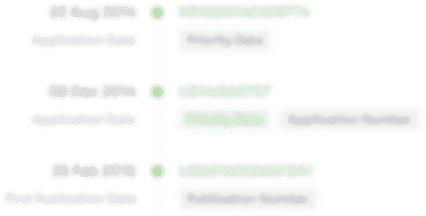
- R&D
- Intellectual Property
- Life Sciences
- Materials
- Tech Scout
- Unparalleled Data Quality
- Higher Quality Content
- 60% Fewer Hallucinations
Browse by: Latest US Patents, China's latest patents, Technical Efficacy Thesaurus, Application Domain, Technology Topic, Popular Technical Reports.
© 2025 PatSnap. All rights reserved.Legal|Privacy policy|Modern Slavery Act Transparency Statement|Sitemap|About US| Contact US: help@patsnap.com