tvf-emd-mcqrnn load probability prediction method based on fuzzy c-means clustering
A TVF-EMD-MCQRNN, mean clustering technology, applied in forecasting, neural learning methods, character and pattern recognition, etc., can solve the problems of fuzzy, limited, preprocessing, etc., the impact of load accuracy, and improve forecasting efficiency and efficiency. Accuracy, avoiding quantile crossover problems, and predicting the effect of accurate results
- Summary
- Abstract
- Description
- Claims
- Application Information
AI Technical Summary
Problems solved by technology
Method used
Image
Examples
Embodiment Construction
[0036] In this embodiment, a TVF-EMD-MCQRNN load probability prediction method based on fuzzy C-means clustering, such as figure 1 As shown, proceed as follows:
[0037] Step 1. Obtain the power load data and its influencing factors and perform preprocessing to obtain the preprocessed dataset Dataset={[G m (t), P(t)]|t=1,2,...,T; m=1,2,...,M}, including: power load after pretreatment {P(t)|t =1,2,...,T} and M influencing factors of electric load {G m (t)|m=1,2,...,M; t=1,2,...,T}, where P(t) and G m (t) are respectively the power load at the tth time point and the mth influencing factor at the corresponding tth time point; T' represents the number of time points, and M represents the number of types of power load influencing factors;
[0038] Step 2. Set the time interval as s time points, and group the preprocessed data set Dataset to obtain I group of sample data, and I satisfies [T′ / s], where the i-th group of sample data is expressed as Dataset i =[G' m (i), P'(i)], ...
PUM
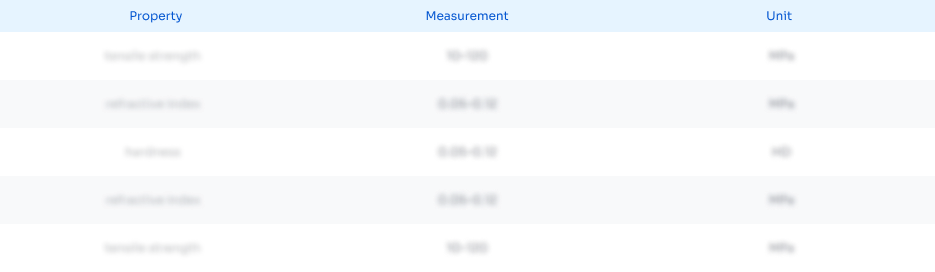
Abstract
Description
Claims
Application Information
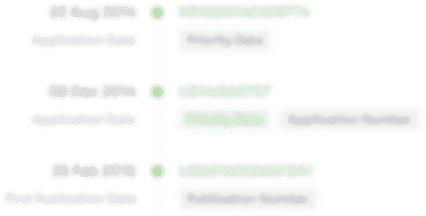
- R&D Engineer
- R&D Manager
- IP Professional
- Industry Leading Data Capabilities
- Powerful AI technology
- Patent DNA Extraction
Browse by: Latest US Patents, China's latest patents, Technical Efficacy Thesaurus, Application Domain, Technology Topic, Popular Technical Reports.
© 2024 PatSnap. All rights reserved.Legal|Privacy policy|Modern Slavery Act Transparency Statement|Sitemap|About US| Contact US: help@patsnap.com