Diabetic retinopathy classification method based on multi-scale convolutional neural network
A technology of convolutional neural network and diabetic retina, which is applied in the field of medical image processing, can solve the problems of only focusing on and difficult to achieve accurate detection at the same time, and achieve the effect of improving performance, realizing simultaneous detection and accurate detection
- Summary
- Abstract
- Description
- Claims
- Application Information
AI Technical Summary
Problems solved by technology
Method used
Image
Examples
Embodiment 1
[0027] Embodiment 1: The diabetic retinopathy classification method based on multi-scale convolutional neural network comprises the following steps:
[0028]Step 1. The training of the convolutional neural network model is inseparable from the support of sufficient quantity and high-quality training and test data sets. Therefore, this embodiment establishes a high-resolution fundus image data set with pixel-accurate diabetic retinopathy markers ( NJU_DR), for model training and testing. The dataset includes 100 color fundus images, of which 84 belong to the training set and 16 belong to the test set. The fundus images of this data set cover retinal images of various degrees of lesions from mild non-proliferative diabetic retinopathy to proliferative diabetic retinopathy, and have ultra-high resolution images of 3800 (±100) x 2900 (±200) pixels resolution. All retinal images are captured by a Daytona fundus camera with a field of view of 200 degrees, which is the leading fund...
PUM
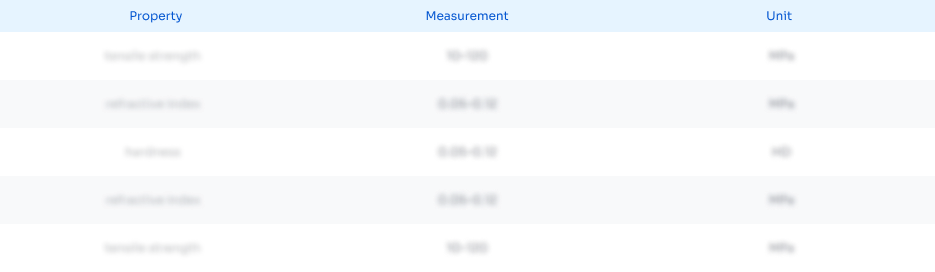
Abstract
Description
Claims
Application Information
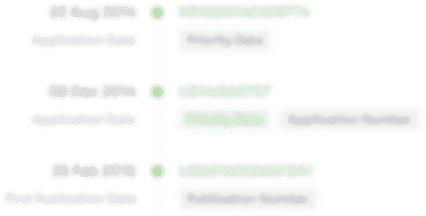
- R&D
- Intellectual Property
- Life Sciences
- Materials
- Tech Scout
- Unparalleled Data Quality
- Higher Quality Content
- 60% Fewer Hallucinations
Browse by: Latest US Patents, China's latest patents, Technical Efficacy Thesaurus, Application Domain, Technology Topic, Popular Technical Reports.
© 2025 PatSnap. All rights reserved.Legal|Privacy policy|Modern Slavery Act Transparency Statement|Sitemap|About US| Contact US: help@patsnap.com