Effective training method based on PCB noise annotation data
A technology for labeling data and training methods, which is applied in image data processing, instruments, character and pattern recognition, etc., and can solve problems such as small distribution, long time consumption, and difficulty in manual detection
- Summary
- Abstract
- Description
- Claims
- Application Information
AI Technical Summary
Problems solved by technology
Method used
Image
Examples
Embodiment Construction
[0069] The technical solutions of the present invention will be further described below in conjunction with the accompanying drawings and embodiments.
[0070] The object of the present invention is to provide a kind of effective training method based on PCB noise labeling data, and its feature is to collect image at first, set up data set, comprise the PCB image data set (Dataset1) that contains a large amount of noise labels and PCB image data through data cleaning Set (Dataset2); then extract the features of each image in the image dataset through the integrated Efficientnet model; then reduce the dimensionality of the extracted features through a deep convolutional network; then use the cosine loss function to train the classifier model; finally use transfer learning Alternately train the two data sets, and fine-tune on the images with large resolution in Dataset2, and finally realize the effective training of the data. This method is mainly used to solve the problem of im...
PUM
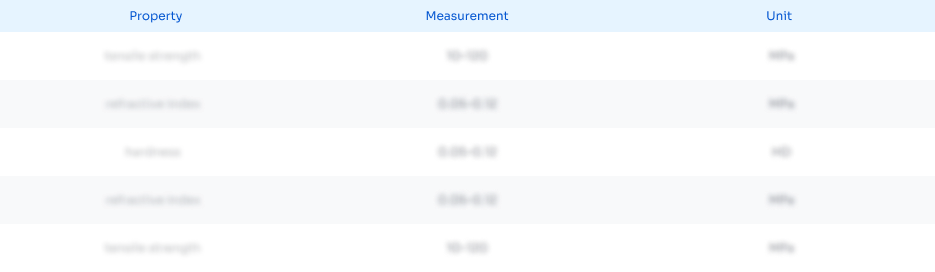
Abstract
Description
Claims
Application Information
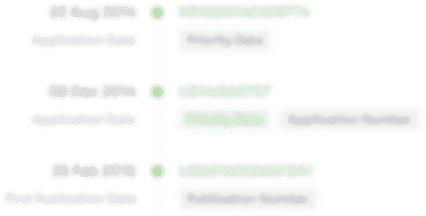
- R&D
- Intellectual Property
- Life Sciences
- Materials
- Tech Scout
- Unparalleled Data Quality
- Higher Quality Content
- 60% Fewer Hallucinations
Browse by: Latest US Patents, China's latest patents, Technical Efficacy Thesaurus, Application Domain, Technology Topic, Popular Technical Reports.
© 2025 PatSnap. All rights reserved.Legal|Privacy policy|Modern Slavery Act Transparency Statement|Sitemap|About US| Contact US: help@patsnap.com