Multi-label text classification method based on statistics and pre-trained language model
A language model and text classification technology, applied in the direction of text database clustering/classification, semantic analysis, character and pattern recognition, etc., can solve the problems of manual design, great influence of classification effect, and high cost of labeling data set acquisition. The effect of improving accuracy
- Summary
- Abstract
- Description
- Claims
- Application Information
AI Technical Summary
Problems solved by technology
Method used
Image
Examples
Embodiment Construction
[0042] The technical solution of the present invention will be further described below in conjunction with the accompanying drawings.
[0043] Such as figure 1 As shown, a kind of multi-label text classification method based on statistics and pre-trained language model of the present invention comprises the following steps:
[0044] S1. Preprocess the training corpus that needs to be classified; the specific implementation method is: obtain the corpus data set OrgData that needs to be labeled, and remove stop words (such as stop words such as "le", "a" and special symbols, etc. words), and then get NewData and save it.
[0045] S2. Establish a label acquisition model based on statistical methods and language models; the label acquisition model includes sequentially connected keyword layers, input coding layers, pre-trained language model layers, and similarity analysis layers, such as figure 2 shown.
[0046] Keyword layer: obtain the top k keywords by statistical methods ...
PUM
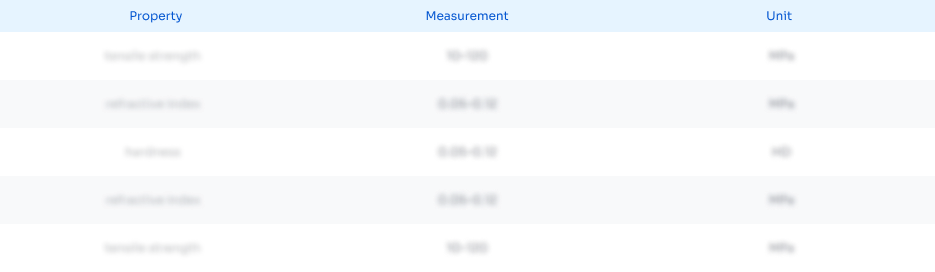
Abstract
Description
Claims
Application Information
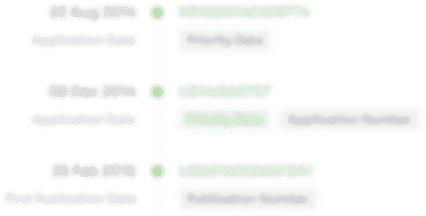
- R&D Engineer
- R&D Manager
- IP Professional
- Industry Leading Data Capabilities
- Powerful AI technology
- Patent DNA Extraction
Browse by: Latest US Patents, China's latest patents, Technical Efficacy Thesaurus, Application Domain, Technology Topic, Popular Technical Reports.
© 2024 PatSnap. All rights reserved.Legal|Privacy policy|Modern Slavery Act Transparency Statement|Sitemap|About US| Contact US: help@patsnap.com